MotionDiffuse: Create human-controlled motion with text with diffusion modeling
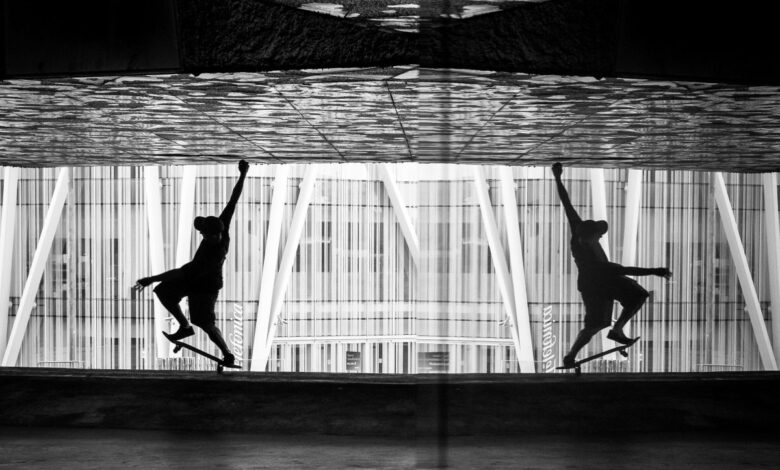
Human movement pattern very important in creating animated virtual characters. However, current approaches require complex equipment and field experts. A recent article on arXiv.org recommends MotionDiffuse, a flexible and controllable motion creation framework that can create varied movements with comprehensive texts.

Human movement – an abstract artistic figure. Credit: Boris Thaser via Pxherefree license
The researchers were inspired by text conditioning image generation and propose to incorporate the Acoustic Diffusion Probability Model into motion generation. Multimodal linear transformer is proposed to achieve motion synthesis with arbitrary length depending on motion duration. Furthermore, MotionDiffuse handles detailed text descriptions that help to mobilize the whole and time-varying signals.
The review shows that the proposed approach achieves a modern state on two conditioned motion generation tasks.
Human motion modeling is important for many modern graphics applications, which often require professional skills. To remove skill barriers for lay people, recent motion-generating methods can directly produce human movement that is tuned based on natural language. However, there are still many challenges to achieving rich and detailed motion creation with different text inputs. To solve this problem, we propose MotionDiffuse, the first diffusion model-based text-driven motion generation framework, which exhibits several desirable properties compared to existing methods. 1) Probability mapping. Instead of a deterministic language-motion mapping, MotionDiffuse generates movements through a series of steps that reduce variation in which variations are introduced. 2) Synthesize reality. MotionDiffuse excels at modeling complex data distributions and creating dynamic motion sequences. 3) Multi-level operation. MotionDiffuse responds to detailed body part instructions and motion synthesis of arbitrary length with time-varying text prompts. Our tests show that MotionDiffuse outperforms existing SoTA methods by convincingly profitable text-driven motion generation and action-conditioned motion generation. A qualitative analysis further demonstrates MotionDiffuse’s controllability to produce full motion. Home page: This https URL
Research articles: Zhang, M., “MotionDiffuse: Text-Driven Human Movement Generation with Diffusion Modeling”, 2022. Links: https://arxiv.org/abs/2208.15001