How AI and machine learning can predict disease and promote health equity
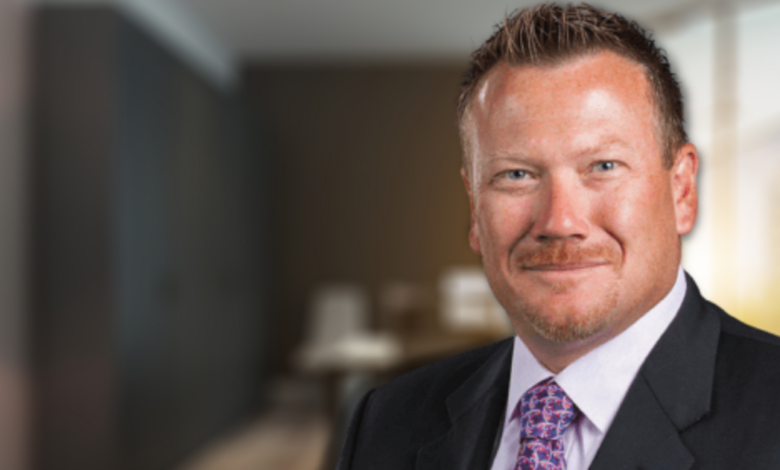
Artificial intelligence and machine learning are key to unlocking patient data and solving some of healthcare’s most complex problems. Even as the United States seeks to put the COVID-19 pandemic in the rearview mirror, many survivors of the initial illness suffer debilitating long-term health effects, especially those with underlying medical conditions.
Technology allows easier access to different data sources without compromising data privacy or integrity. Additionally, advanced analytics provide real-time insights, enabling providers to predict outcomes and diagnose disease early to intervene with patients at long-term risk of COVID-19. and other chronic diseases.
To dig deeper into these technologies and their ramifications in healthcare, visit Healthcare IT News spoke with Brett Furst, president of HHS Tech Group.
Q. How can health systems IT leaders leverage AI and machine learning to unlock and explore patient data for early detection and accurate treatment of chronic disease?
ONE. Health systems IT leaders face two key challenges specific to healthcare data: It’s cluttered and there’s a significant amount of data.
For example, much of a patient’s valuable information regarding social determinants of health is buried as unstructured data in the notes section of an electronic health record, making it difficult to for capture and analysis. Furthermore, in addition to patient data in EHRs, a great deal of information can be gathered from other sources, such as surveys, games, retail, and social media, which in many cases have never been reported before. fit.
When researchers and providers combine diverse data sets, the healthcare industry can gain new insights at the population health level. However, due to the clutter and volume of healthcare data, manual or human efforts to derive value from that data are not enough, and that’s where artificial intelligence and machine learning come in. join the picture.
Artificial intelligence, machine learning, and other related technologies enable vendors and researchers to gain greater insights in a significantly shorter time frame than extraction, collection, and Traditional data analysis.
Artificial intelligence and machine learning are essential tools for solving complex healthcare data problems, such as identifying patients with signs of undiagnosed chronic disease or analyzing them. wastewater samples to identify the next pandemic.
H. The nation’s largest COVID-19 research database and related analytics help health system leaders and other organizations like medical associations better understand the impact of COVID-19 How about behavioral health and populations with underlying chronic diseases?
ONE. The COVID-19 research database has a wide array of data on most of the US population, including statements, meetings, social determinants of health, and many other types of clinical data. The database efficiently brings together these huge data sets in one place, linking them and driving discoveries.
For example, on the impact of pre-existing chronic conditions, such as diabetes, chronic obstructive pulmonary disorder, and chronic kidney disease, on patients infected with COVID-19.
By leveraging one of the world’s most comprehensive cross-linked datasets, researchers can draw new conclusions about how COVID-19 affects vulnerable populations. For example, researchers can study data on patients’ access to health care, nutrition, and economic opportunity, and see how COVID-19 infection has affected individuals individuals in these groups.
Ultimately, the goal is to establish a causal relationship between multiple variables to determine how they interact with and affect people with COVID-19. But before that can be achieved, we need to derive correlations from billions of health records, which requires heavy use of artificial intelligence, machine learning, and advanced analytics.
Q. How do artificial intelligence and machine learning predict health outcomes and drive the adoption of evidence-based strategies to improve the quality and safety of treatment?
ONE. Providers are using artificial intelligence and machine learning to identify groups at risk for chronic conditions such as chronic kidney disease and hypertension before these patients are diagnosed and health status Theirs becomes more serious.
Although patients in these populations are underdiagnosed, predictive modeling can take into account multiple data factors to accurately identify patients who require intervention. For example, consider chronic kidney disease, a common, serious, costly, and often preventable disease. However, many people in the early stages of the disease do not even realize they have the disease because patients often show few symptoms at first.
At the same time, providers can use AI and machine learning to measure the effectiveness of existing interventions for patients at different stages of chronic kidney disease. Coupled with real-time analytics that provide insights that help identify patients most at risk for chronic kidney disease, these technologies empower healthcare providers to serve patients. their best with more timely diagnosis and treatment.
Q. How can AI and machine learning be used to close gaps in health equity and improve access to care for underserved populations?
ONE. The first step to closing gaps in health equity and improving access to care for underserved populations is to identify patients who will benefit from these initiatives. this.
For example, we can accurately identify many different patient groups, such as those without transportation, those who live in food deserts, or those who live far from a healthcare facility nearest more than 10 miles. Once identified, we can refer patients to community-based organizations that address their specific SDOH needs.
In addition, local governments can change the infrastructure, such as using empty parking lots to set up mobile testing laboratories in areas where people have difficulty accessing the epidemic. care service. Likewise, governments can work with local retailers, such as discount stores, once we identify scarce food sources to stock up on food options. fresh.
By combining geographic map data with patient SDOH and demographic data, providers can discover where disparities exist and work with local stakeholders to design programs to address those disparities.
Follow Bill’s HIT coverage on LinkedIn: Bill Siwicki
Email the writer: [email protected]
Healthcare IT News is a publication of HIMSS Media.