Uncertainty Of Measurement of Routine Temperatures–Part Three – Watts Up With That?
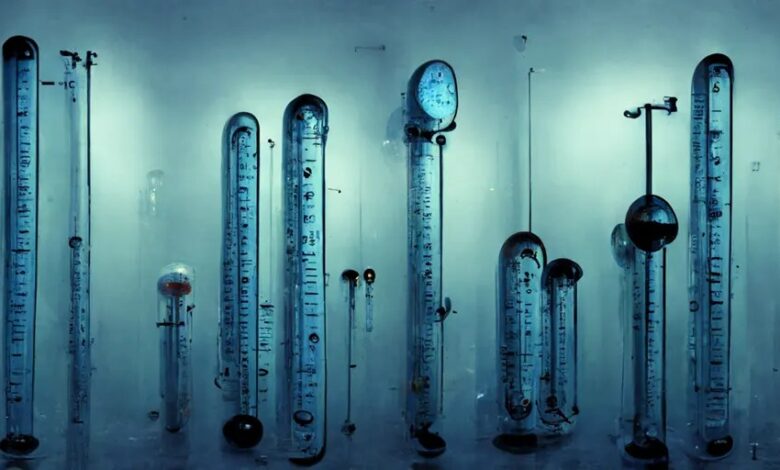
By Thomas Berger and Geoffrey Sherrington.
…………………………………………………..
Please welcome co-author Tom Berger, who has studied these Australian temperature data sets for several years using mathematical forensic investigation methods. Many are based around the statistics software SAS JMP.
Please persist with reading this long essay to the end, because we are confident that there is a mass of new material that will cause you to rethink the whole topic of the quality of fundamental data behind the climate change story. I found it eye-opening, starting with this set of temperatures from the premier BOM site at Sydney Observatory. Supposedly “raw” data are not raw, because identical data are copied and pasted from July 1914 to July 1915.
This has happened before the eyes of those who created the homogenised version 22 of ACORN-SAT, released late in 2021. It signalled the opening of a Pandora’s Box, because this is not the only copy and paste in the Sydney “raw” data. See also June 1943 to June 1944 (whole month), December 1935 to December 1936 (whole month, but 2 values missing in 1935 have values in 1936).
Tom has argued to me that –
“The strategy of getting people to respect the scientific way is a lost cause I am afraid. The idea is to show various things and let them come to the conclusion by themselves!!! This is vital.”
This goes against my grain as a scientist for many years, but doing what open-minded scientists should do, we shall accept and publicise Tom’s work. Tom is suggesting that we go more towards countering propaganda, so let us do that by starting with the topic of “raw” temperature measurements. This leads to what “adjusted” temperatures can do to intellectual and mathematical purity. Then Tom will introduce some methodologies that we are confident few readers have seen before.
For these temperatures, “raw” data is what observers write down about their daily observations. I have visited the Library of the Bureau of Meteorology in Melbourne and with permission, have photographed what was shown as “raw” data. Here is one such sheet, from Melbourne, August 1860. (I have lightly coloured the columns of main interest.)
http://www.geoffstuff.com/melborig.jpg
The next month, September 1860, has quite different appearance, suggesting monthly tidy-up processes.
http://www.geoffstuff.com/nextmelborig.jpg
Those who have taken daily measurements might feel that these sheets are transcriptions from earlier documents. I do. The handwriting changes from month to month, not day to day. Transcriptions are fertile ground for corrections.
Apart from adjustments to raw data, “Adjusted” data as used in this essay almost always derives from the ACORN-SAT homogenisation process used by BOM. This has four versions named in shorthand as V1, V2, v21 and v22 for Version 1, version 2. Version 2.1 and version 2.2. Maximum daily temperatures and minima are abbreviated as graph labels in the style minv22 or maxraw, to show but 2 examples. Most of the weather stations are named by locality like nearest town, with the ACORN-SAT stations listed in this catalogue.
http://www.bom.gov.au/climate/data/acorn-sat/stations/#/23000
The ACORN-SAT adjustment/homogenisation process is described in several BOM reports such as these and more –
http://www.bom.gov.au/climate/change/acorn-sat/documents/About_ACORN-SAT.pdf
http://www.bom.gov.au/climate/data/acorn-sat/documents/ACORN-SAT_Report_No_1_WEB.pdf
Without the truly basic, fundamental, raw evidence such as original observer sheets that I have sought without success, I now turn to Tom for his studies of available data to see what more is found by forensic examination.
………………………………………………………………………..
Australian Climate Data used for creating trends by BOM is analysed and dissected. The results show the data to be biased and dirty, even up to 2010 in some stations, making it unfit for predictions or trends.
In many cases the data temperature sequences are strings of duplicates and replicated sequences that bear no resemblance to observational temperatures.
This data would be thrown out in many industries such as pharmaceuticals and industrial control. Many of the BOM data handling methodologies are unfit for most industries.
Dirty data stations appear to have been used in the network to counter the scarcity of the climate network argument made against the Australian climate network. (Modeling And Pricing Weather-Related Risk, Antonis K. Alexandridis et al)
We use a forensic exploratory software (SAS JMP) to identify plausibly fake sequences, but also develop a simple technique to show clusters of fabricated data. Along with Data Mining techniques, it is shown causally that BOM adjustments create fake unnatural sequences that no longer function as observational or evidential data.
“These (Climate) research findings contain circular reasoning because in the end the hypothesis is proven with data from which the hypothesis was derived.”
Circular Reasoning in Climate Change Research – Jamal Munshi
BEFORE WE BEGIN – AN ANOMALY OF AN ANOMALY.
A persistent myth:
“Note that temperature timeseries are presented as anomalies or departures from the 1961–1990 average because temperature anomalies tend to be more consistent throughout wide areas than actual temperatures.” –BOM (link)
This is nonsense. Notice the weasel word “tend” which isn’t on the definitive NASA web site. BOM is attempting to soften the statement by providing an out. Where weasel words such as “perhaps”, “may”, “could”, “might” or “tend” are used, these are red flags that provide useful areas for investigation.
Using an arbitrarily chosen average of a 30 year block of temperatures, an offset, does not make this group “normal”, nor does it give you any more data than you already have.
Plotting deviations from this arbitrarily chosen offset, for a limited network of stations gives you no more insight and it most definitely does not mean you can extend analysis to areas without stations, or make extrapolation any more legitimate, if you haven’t taken measurements there.
Averaging temperature anomalies “throughout wide areas” if you only have a few station readings, doesn’t give you any more an accurate picture than averaging straight temperatures.
THINK BIG, THINK GLOBAL:
Looking at Annual Global Temperature Anomalies: Is this the weapon of choice when creating scare campaigns? It consists of averaging nearly a million temperature anomalies into a single number. (link)
Here a summary graph from the BOM site dated 2022 (data actually only to 2020).

The Wayback website finds the years 2014 and 2010 and 2022 from the BOM site. Nothing earlier was found. Below is the graph compiled in year 2010.



Looking at the two graphs you can see differences. These infer that there has been warming, but by how much?
Overlaying the temperature anomalies compiled in 2010 and 2020 helps.



BOM emphasise that their adjustments and changes are small, for example:
“The differences between ‘raw’ and ‘homogenised’ datasets are small, and capture the uncertainty in temperature estimates for Australia.” -BOM (link)
Let’s test this with a hypothesis: Let’s say that every version of the Global Temperature Anomalies plots (2010, 2014, 2020) has had warming added significantly at the 95% level (using the same significance levels as BOM).
The null or zero hypothesis is that the distributions are the same, no significant warming has taken place between the various plots.
Therefore, 2010 > 2014 <2020.
To test this we use:
A NONPARAMETRIC COMBINATION TEST.
This is a permutation test framework that allows accurate combining of different hypotheses. It makes no assumptions besides the observations being exchangeable, works with small samples and is not affected by missing data.
Pesarin popularised NPC, but Devin Caughey of MIT has the most up to date and flexible version of the algorithm, written in R. (link). It is also commonly used where a large number of contrasts are being investigated such as in brain scan labs. (link)
“Being based on permutation inference, NPC does not require modelling assumptions or asymptotic justifications, only that observations be exchangeable (e.g., randomly assigned) under the global null hypothesis that treatment has no effect. ” — Devin Caughey, MIT
After running NPC in R, our main result:
2010<2014 results in a p value = 0.0444
This is less than our cut-off of p value = 0.05 so we reject the null and can say that the Global Temperature Anomalies in the plots 2010 and 2014 have had warming significantly added to the data, and that the distributions are different.
The result of 2020 > 2014 has a p-value = 0.1975
We do not reject the null here, so 2014 is not significantly different from 2020.
If we combine p-values using hypothesis (2010<2014>2020 i.e. increases in warming in every version) with NPC, we get a p-value of 0.0686. This just falls short of our 5% level of significance, so we don’t reject the null, although there is evidence supporting progressive warming on the plots.
The takeaway here is that Global Temperature Anomalies plots have been significantly altered by warming up temperatures between the years 2010 and 2014, after which they stayed essentially similar. The question is, do we have an actual warming or a synthetic warming?
I SEE IT BUT I DON’T BELIEVE IT….
” If you are using averages, on average you will be wrong.” — Dr. Sam Savage on The Flaw Of Averages
As shown below, the BOM have a propensity to copy/paste or alter temperature sequences, creating blocks of duplicate temperatures or duplicate sequences lasting a few days or weeks or even a full month. They surely wouldn’t have done this with a small sample such as these Global Temperature Anomalies, would they?



Incredibly, there is a duplicate sequence even in this small sample. SAS JMP calculates the probability of seeing this at random given this sample size and number of unique values, is equal to seeing 10 heads in a row in a coin flip sequence. In other words, unlikely, but possible. For sceptics, more likely this is the dodgy data hypothesis.
Dodgy sequences exist in raw data when up to 40 stations are averaged, they are also created by “adjustments”.
THE CASE OF THE DOG THAT DID NOT BARK
Just as the dog not barking on a specific night was highly relevant to Sherlock Holmes in solving a case, so it is important with us knowing what is not there.
We need to know what variables disappear and also which ones suddenly reappear.
“A study that leaves out data is waving a big red flag. A
decision to include or exclude data sometimes makes all the difference in the world.” — Standard Deviations, Flawed Assumptions, Tortured Data, and Other Ways to Lie with Statistics, Gary Smith.



A summary of missing data from Palmerville weather station in North Queensland shows the process of how data is deleted or imputed by various versions of BOM software. Looking at minimum temps, the initial data the BOM works with is raw, so minraw has 4301 missing temps, then after minv1 adjustments there are now 4479 temps missing, a loss of 178 values.
After version minv2 adjustments, there are now 3908 temps missing, so now 571 temps have been imputed or infilled.
A few more years later technology has sufficiently advanced for BOM to bring out a new minv21 and now there are 3546 temps missing — a net gain of 362 temps that have been imputed/infilled. By version minv22 there are 3571 missing values and 25 values now go missing in action.
Keeping in mind BOM had no problem with massive data imputation of 37 years as in Port Macquarie, where no data existed, data was created along with outliers and trends and fat tailed months (no doubt averaged from the 40 station shortlist the BOM software looks for). It’s almost as if the missing/added in values help the hypothesis.
Data that is missing NOT at random is commonly called MNAR (missing not at random) and it creates a data bias.
Like this from Moree station, NSW:



One third of the time series disappears on a Sunday! The rest of the week the values are back. Version minv1 is permanently deleted – apparently after the raw was created, BOM didn’t like the first third of the data for minv1 and deleted it. Then they changed their minds with all the other versions and created data – except for Sunday, of course.
Mildura likes to be different, though, and has Monday as the magically disappearing data day:



Nhill on a Sunday (below left) is different to a Monday (below right)…..



Which is different to Tuesday – Saturday (below). Although, if you notice, there is still a slice of missing data at around 1950. But why push a good thing with all this data imputation; leaving some gaps makes it all look more authentic.



MISSING TEMPERATURE RANGES.
Scatterplots are amongst the first things we do with exploratory data work. The first thing you notice with BOM data is that things go missing, depending on the month or decade or day of the week. Complete ranges of temperature can go missing for many, even most of the 110-year ACORN-SAT time series.
Palmerville for example:



The long horizontal gaps or “corridors” of missing data show complete ranges of temperatures that do not exist for most of the time series. Here, it takes till about 2005 to get all the temperature ranges to show. The other thing to notice is that adjustments that follow the raw, make things worse.



Above – same for November.



The disturbing trend here is that adjustments often make things worse — data goes missing, temperature ranges go missing, fake sequences are introduced:



Looking at the raw, which came first, you can see how a duplicated sequence came to be after “adjustments”. The result is an obviously tampered temperature sequence.
In the Charleville example below, comparing 1942 to 1943, we can see single raw value in 1942 being deleted from 1943 and a single minv2 value being changed slightly between the years …. but a long duplicated sequence is being left as it was.



There are two types of sequences of interest here:
1 – A run of duplicated temperatures.
2 – A sequence of temperatures that is duplicated somewhere else.
There are hundreds and hundreds of these sequences over most of the ACORN-SAT time series. Sydney even has two and a half months copy/pasted into another year, as we have seen. Computers do not select “random” data that is just one calendar month long, again and again – people do that.
The probability of seeing these sequences at random are calculated by SAS JMP software to be from unlikely to impossible. Even simulating data with auto correlation using Block Bootstrap methodology shows the impossibility of the sequences.
The result are fake temperature runs which fail most observational data digit tests, such as Benford’s Law for anomalies, Simonsohn’s Number Bunching tests (www.datacolada.com), and Ensminger+Leder-Luis bank of digit tests (Measuring Strategic Data Manipulation: Evidence from a World Bank Project By Jean Ensminger and Jetson Leder-Luis)
Palmerville examples are below. The left hand duplicated sequences are a very devious way of warming — a warmer month sequence is copied into a colder month.
BOM is obviously having trouble getting clean data even in 2004.



Palmerville ranks among the worst Acorn sites for data purity. Very many of the temperature observations are integers, in whole numbers, nothing after the decimal. From the ACORN-SAT station catalogue,
“The same observer performed observations for 63 years from 1936 to 1999, and was the area’s sole remaining resident during the later part of that period. After manual observations ceased upon on her departure in 1999, observations did not resume until the automatic station was commissioned.”
The stations at Melbourne Regional (1856-2014 approx) and Sydney Observatory (1857-Oct 2017 approx) are assumed here to represent the highest of BOM quality. (link)
Temperature ranges go missing for many years too, they just never appear until the 2000’s in many cases. And this can happen after adjustments as well.
Below – Let’s stay with Palmerville for all the August months from 1910 to 2020. For this we will use the most basic of all data analysis graphs, the scatterplot. This is a data display that shows the relationship between two numerical variables.



Above — This is a complete data view (scatterplot) of the entire time series, minraw and minv22. Raw came first in time (bottom in red) so this is our reference. After adjustments from minv22, entire ranges have gone missing, the horizontal “gutters” show missing temperatures that never appear. Even at year 2000 you see horizontal gaps where decimal values have disappeared, so you only get whole integer temps such 15C, 16C and so on.
The BOM station catalogue notes that the observer departed in 1999 and for the AWS “The automatic weather station was installed in mid-2000, 160 m south-east of the former site, but did not begin transmitting data until July 2001.” So, there seems to have been no data collected between these dates, From whence did it come, to show in red on the graph above?
Raw has been adjusted 4 times with 4 versions of state-of-the-art BOM software and this is the result – a worse outcome.






March data is also worse after adjustments. They had a real problem with temperature from around 1998-2005.
Below — Look at before and after adjustments. These are very bad data handling procedures and it’s not random, so don’t expect this kind of manipulation to cancel errors out.



SUNDAY AT NHILL = MISSING DATA NOT AT RANDOM
A BIAS IS CREATED WITH MISSING DATA NOT AT RANDOM.(LINK).
Below – Nhill on a Saturday has a big chunk of data missing in both raw and adjusted.



Below: Come Sunday, voila …. thousands of raw temperatures now exist, but adjusted data is still missing.



Below – Wait, there’s more – now it’s Monday, and just like that, thousands of adjusted temperatures appear!



Below — Mildura on a Friday.



Below — Mildura on a Sunday:



Below — Mildura on a Monday:



Above – On Monday, a big chunk disappears in adjusted data. Strangely the thin strip of missing raw data at around 1947 is infilled in minv2.2.
This kind of data handling is indicative of many other problems of bias.
SYDNEY DAY-OF-WEEK EFFECT.
Taking all the September months in the Sydney time series from 1910-2020 shows Friday to be at a significantly different temperature than Sunday and Monday.
The chance of seeing this at random is over 1000-1:



Saturday is warmer than Thursday in December too, this is highly significant.



NEVER ON A SUNDAY.
Below — Moree on a Monday to Saturday looks like this.



Below — But then Sunday in Moree happens, and a third of the data disappears! (except for a few odd values).



A third of the time series goes missing on Sunday! It seems that the Greek comedy film Never On A Sunday with Greek prostitute Ilya attempting to relax Homer (but never on a Sunday) has rubbed off onto Moree.
ADJUSTMENTS CREATE DUPLICATE SEQUENCES OF DATA
Below — Sydney shows how duplicates are created with adjustments:
The duplicated data is created by the BOM with their state-of-the-art adjustment software, they seem to forget that this is supposed to be observational data. Different raw values turn into a sequence of duplicated values in maxv22!



A SLY WAY OF WARMING:
Last two examples from Palmerville, one showing a devious way of warming by copying from March and pasting into May!






“Watch out for unnatural groupings of data. In a fervent quest for publishable theories—no matter how implausible—it is tempting to tweak the data to provide more support for the theory and it is natural to not look too closely if a statistical test gives the hoped-for answer.”
— Standard Deviations, Flawed Assumptions, Tortured Data, and Other Ways to Lie with Statistics, Gary Smith.
“In biased research of this kind, researchers do not objectively seek the truth, whatever it may turn out to be, but rather seek to prove the truth of what they already know to be true or what needs to be true to support activism for a noble cause (Nickerson, 1998).”
— Circular Reasoning In Climate Change Research, Jamal Munshi
THE QUALITY OF BOM RAW DATA
We shouldn’t be talking about raw data, because it’s a misleading concept……
“Reference to Raw is in itself a misleading concept as it often implies some pre-adjustment dataset which might be taken as a pure recording at a single station location. For two thirds of the ACORN SAT there is no raw temperature series but rather a composited series taken from two or more stations.” — the BOM
“Homogenization does not increase the accuracy of the data – it can be no higher than the accuracy of the observations.” (M.Syrakova, V.Mateev, 2009)
The reason it’s misleading is because BOM continues to call the data “raw” when it’s a single average of many stations; the default shortlist on the BOM software is 40 stations. This is the weak form of the Flaw of Averages (Dr. Sam Savage, 2009), so this single composite number is likely to be wrong.
At the data exploratory stage, one of the first things to do is at the distribution with a histogram. This is where the problems start:









The repeats/frequency of occurrence is on the Y axis, the raw temps are on the X axis. The histogram shows you how often certain temperatures occur. It is immediately evident that there is a problem; there appear to be two histograms overlayed on each other. The spiky one is very methodical with even spacing. It’s soon obvious this is not clean observational data.
It tuns out that the cause of these spikes is Double Rounding Imprecision where Fahrenheit is rounded to say 1 degree precision, then converted to Celsius and rounded to 0.1 C precision creating an excess of decimal 0.0’s and a scarcity of 0.5’s (with this example); different rounding scenarios exist where different decimal scarcities and excesses were created in the same time series!
The below image comes from the referenced paper and shows various rounding/converting/rounding protocols. For instance, looking at the first set of numbers, 0.5C and 2.0F, this scenario means that Fahrenheit was rounded to 2 degrees precision, converted then rounded to 0.5C precision. All these scenarios were investigated in the paper, but what affects the BOM data specifically is the 6th from the bottom protocol – rounding to 1.0F precision, converting and then rounding to 0.1C precision. This causes excess decimal 0’s and no 0.5’s



The paper–
“Decoding The Precision Of Historical Temperature Observations” — Andrew Rhimes, Karen A McKinnon, Peter Hubers.
The BOM Double Rounding Errors that exist are caused by this particular protocol, and it can easily be seen below when decimal frequency per year is compared to years. The decimal frequency per year use is key to seeing the overall picture:



This shows Yamba and clearly shows a shortfall or scarcity of 0.5 decimal use from 1910-1970, and the excess use of 0.0 and 0.5 decimals from 1980-2007 or so. It’s obvious looking at most country stations that decimal problems exist long after decimalisation in the 70’s, and in some cases problems exist up to 2010.
Look at Bourke below for example.



These different Double Rounding Error scenarios put records in doubt in some cases (see above paper), as well as causing the time series to be out of alignment. As can be seen, BOM has not corrected the Double Rounding Error problem, even though simple Matlab correction software exists within the above paper.
Marble Bar, below, shows the exact same problem, uncorrected Double Rounding Errors creating a scarcity of 0.5 decimal use. Again, at around 2000 there are an excess 0.0 and 0.5 decimals in use. From the BOM station catalogue for Marble Bar,
“The automatic weather station opened in September 2000, with instruments in the same screen as the former manual site (4020). The manual site continued until 2006 but was only used in ACORN-SAT until the end of 2002 because of deteriorating data quality after that.”



Getting back to the spiky histograms, here is how they are formed:



Using SAS JMP dynamically linked data tables, selecting specific data points, links these selections with all the other data tables, showing how the spikes are formed—
The scarcity of 0.5 decimals creates a lower level histogram (see above plot) whereby the data points drop down to a lower level, leaving spaces and spikes. This is causing the strange looking histograms and is a tip off that the data are not corrected for Double Rounding Errors. Virtually all BOM country stations examined have this problem.
ADJUSTMENTS, OR TWEAKING TEMPERATURES TO INCREASE TRENDS.
“For example, imagine if a weather station in your suburb or town had to be moved because of a building development. There’s a good chance the new location may be slightly warmer or colder than the previous. If we are to provide the community with the best estimate of the true long-term temperature trend at that location, it’s important that we account for such changes. To do this, the Bureau and other major meteorological organisations such as NASA, the National Oceanic and Atmospheric Administration and the UK Met Office use a scientific process called homogenisation.” — BOM
The condescending BOM quote under the header prepares you for what is coming:
A whole industry has been created in adjusting “biases”. BOM has used the SNHT algorithm with a default list of 40 shortlisted stations to average into a single number. Using a significance level of 95% means that 1 in 20 “steps” or “biases” is a false positive.
Using different versions of software in an iterative manner on the same data without using multiplicity corrections to account for luck in finding this “bias”, leads to errors and biases in data.
“Systematic bias as long as it does not change will not affect the changes in temperature. Thus improper placement of the measuring stations result in a bias but as long as it does not change it is unimportant. But any changes in the number and location of measuring stations could create the appearance of a spurious trend.” –Prof Thayer Watkins, San Jose University.
There are no series that can be classified, unequivocally, as homogeneous, so it is not possible to build a perfectly homogenous reference series.
As well, SNHT does not provide any estimate of confidence levels of adjustments.
We are meant to believe that moving a station or vegetation growth or any other bias that is formed leads to these types of adjustments:



A “step change” would look like the arrow pointing at 1940. But look at around 1960–there are a mass of adjustments covering a large temperature range, it’s a chaotic hodge-podge of adjustments at different ranges and years. If we only look at 1960 in Moree:



BOM would have us believe that these chaotic adjustments for just 1960 in this example, are exact and precise adjustments needed to correct biases.
More adjustment scatterplots:






A more likely explanation based on the Modus Operandi of the BOM is that specific months and years get specific warming and cooling to increase the desired trend.
Now looking at the scatterplots you can clearly see that adjustments are not about correcting “step-changes” and biases. Recall, as we saw above, in most cases the adjustments make the data worse by adding duplicate sequences and adding other biases. Bedford’s Law also shows less compliance with adjustments indicating data problems.
Certain months get hammered with the biggest adjustments, and this is consistent with many time series —






And some adjustments depend on what day of the week it is:


















Adjusting by day of week means the Modal Value, the most common temperature in the timeseries, will also be affected by this bias – and hence also the mean temperature.



“Analysis has shown the newly applied adjustments in ACORN-SAT version 2.2 have not altered the estimated long-term warming trend in Australia.” –BOM
“…..pooled data may cancel out different individual signatures of manipulation.” — (Diekmann, 2007)
It is assumed that the early temperatures have the same Confidence Intervals as the more recent temperatures, which is obviously wrong.
Individual station trends can certainly be changed with adjustments, especially with seasons, which BOM software evaluates:






Below we look at values that are missing in Raw but appear in version 2.1 or 2.2.
This tells us they are created or imputed values. In this case the black dots are missing in raw, but now appear as outliers.
These outliers and values, by themselves, have an upward trend. In other words, the imputed/created data has a warming trend (below).



In summary, adjustments create runs of duplicate temperatures, and also create runs of duplicated sequences that exist in different months or years. These are obvious “fake” temperatures – no climate database can justify 17 days (or worse) in a row with the same temp for example.
Adjustments are heavily done in particular months and can depend on what day of the week it is.
Adjustments also cause data to vanish or reappear along with outliers. Data Analysis Digit Tests (Simonsohn’s or Benford’s or Luis-Leder World Bank Digit tests) show that generally the raw data becomes worse after adjustments! As well, complete temperature ranges can disappear even up to 80 years or more.
This data would be unfit for most industries.
THE GERMAN TANK PROBLEM.
In World War II, each manufactured German tank or piece of weaponry was printed with a serial number. Using serial numbers from damaged or captured German tanks, the Allies were able to calculate the total number of tanks and other machinery in the German arsenal.
The serial numbers revealed extra information, in this case an estimate of the entire population based on a limited sample.
This is an example of what David Hand calls Dark Data. This is data that many industries have, never use, but leaks interesting information that can be used. (link)
Now, Dark Data in the context of Australian Climate data would allow us extra insight to what the BOM is doing behind the scenes with the data …. that maybe they are not aware of. If dodgy work was being done, they would not be aware of any information “leakage.”
A simple Dark Data scenario here is very simply done by taking the first difference of a time series. (First differencing is a well-known method in time series analysis).
Find the difference between temperature 1 and temperature 2, then the difference between temperature 2 and temperature 3 and so on. (below)
If the difference between two consecutive days is zero, then the two paired days have the same temperature. This is a quick and easy way to spot paired days that have the same temperature.
Intuition would expect a random distribution with no obvious clumps. Block bootstrap simulations, which preserve autocorrelation, confirm this. For example, some tested European time series have evenly distributed paired days.



Above is deKooy in the Netherlands with a fairly even distribution. Sweden is very similar. Diff0 in the graph refers to the fact that there is zero difference between a pair of temps when using the First Difference technique above, meaning that the 2 days have identical temperatures. The red dots show their spread.
Let’s look at Melbourne, Australia, below:



The paired days with same temperatures are clustered in the cooler part of the graph. They taper out after 2010 or so (fewer red dots). Melbourne data were from different sites, with a change in 2014 approx. from BOM Regional Office site 86071 to the Olympic Park site, 2 km away, 86338.
Below is Bourke, and again you can see clustered red dot data.
From the BOM Station Catalogue –
“The current site (48245) is an automatic weather station on the north side of Bourke Airport … The current site began operations in December 1998, 700 m north of the previous airport location but with only a minimal overlap. These data are used in ACORN-SAT from 1 January 1999.”



Below is Port Macquarie, where there is extremely tight clustering from around 1940-1970.



This data is from adjusted ACORN-SAT sources, not raw data. Varying with adjustments, in many cases there are very large difference before and after adjustments.
The capital cities vary around 3-4% of the data being paired. Country station can go up to 20% for some niche groups.
The hypothesis is this: The most heavily clustered data points are the most heavily manipulated data areas.
Also, some of the red dot clusters can be correlated visually with site changes noted in the catalogue.
Let’s look at a very dense spot at Port Macquarie, 1940-1970.



It is immediately apparent that many days have duplicated sequences. Even though these are shorter sequences, they are still more than you would expect at random, but also note the strange systematic spacing and gaps below.
More from Port Macquarie, BOM station catalogue:
“There was a move 90 m seaward (the exact direction is unclear) in January 1939, and a move 18 m to the southwest on 4 September 1968.The current site (60139) is an automatic weather station at Port Macquarie Airport … The current site (60139) is located on the southeast side of the airport runway. It began operations in 1995 but has only been used in the ACORN-SAT dataset from January 2000 because of some issues with its early data. A new site (60168) was established on the airport grounds in October 2020 and is expected to supersede the current site in due course.”
The example below is for the early 1950s.



Here we have gaps of 1 and 3 between the sequences.
Below we have gaps of 8 between sequences.



Below — now we have gaps of 2, then 3, then 4, then 5. Remember, most time series have many of these “fake” sequences!



Proving Causality
CO2 and warming is based on correlation, but we all know correlation does not mean causation.
Tony Cox is a leading expert in Causal Analysis and has a software toolbox to test causality.
If we use Bourke minimum temperatures over the whole time series, and set as a target “MIN Paired Days, Same Temp”, rules are created by CART to find predictive causal links:
/*Rules for terminal node 3*/
if
MAXpaired days same temp <= 0.06429 &&
MINadjustments > -0.62295 &&
MINadjustments <= -0.59754
terminalNode = 3;
This is saying that if the maximum temp paired days same temp is less than 0.06429 AND adjustments on MIN temps are between -0.62295 AND -.59754, then node 3 is true and a highly predictive cluster of 50% has been found.
NOTE—paired days same temp for MAX series and adjustments by BOM have been picked up as predictive causal!
Port Macquarie MIN temp time series.
Target — MINpaired days same temps
/*Rules for terminal node 3*/
if
MAXadjustments > 0.53663 &&
MAXpaired days same temps <= 0.02329
terminalNode = 3;
class prob= 20%



The ROC curve above for Port Macquarie shows a very predictive model, the most predictive causal variables being MAX paired days same temps with large Max adjustments being causal for predicting MIN Paired Days, Same Temps!
Below is the CART tree output for finding the target in Palmerville:
MIN paired days, same temps.



Here it finds days of the week and years predictive causal. You read the tree by going down a branch If True and read the probability of the cluster being true.
In these cases, and several more that have been tested, the predictive causal result of the target Minimum Paired Days With Same Temps is day of week, year and magnitude of adjustments!
Recall, Paired days, Same Temps were indicative of duplicated or “fake runs” of temperatures. The higher the cluster concentration, the more sequences found.
Clearly the data has serious problems if day of the week is significant during modeling. BOM adjustments are also causal for creating clusters of “fake” sequences.
__________________________________________________
Tom’s part ends here, Geoff resumes.
Here are some questions that arise from Tom’s work.
Why did we have to do this basic quality control investigation of the BOM’s primary raw temperature data in citizen scientist mode?
Was BOM already aware of the large number od cases of corrupted and/or suspicious data, but continued to advise that raw data were essentially untouched except for the removal of typos and a few obvious outliers?
Noting that both “raw” and “ACORN-SAT” data have inherent problems, how can one say that distributions derived from numbers like these have validity?
Therefore, how can one justify a distribution-based argument to allow use of the Central Limit Theorem when so many distributions are tainted?
Can one apply the Law of Large Numbers validly to data that are invented by people and that are not observations?
How does one estimate measurement uncertainty with data that are invented by people?
Where is the Manual for estimation of confidence in imputed values? Is it accurate?
Are observations if this type even fit for purpose?
Why was the BOM given a “free pass” by experts who studied the value of ACORN-SAT? Why did they fail to find data irregularities?
Is it possible to “re-adjust” these millions of observations to ensure that they pass tests of the type described here?
Or should the BOM advise that only data since (say) 1st November 1996 be used in future? (This was when many BOM weather stations changed from manual thermometry to electronic AWS observations).
Are there other branches of observational science that also have problems similar to these, or is the BOM presenting a special case?
We really had to shorten this long essay. Would WUWT readers like to have a Part Four of this series, that shows much more about these temperatures?
IN CONCLUSION.
We do not in any way criticise the many observers who recorded the original temperature data. Our concern is with subsequent modifications to the original data, recalling that Australia’s Bureau of Meteorology (BoM) has a $77million Cray XC-40 supercomputer named Australis. One day of keystrokes on Australis can plausibly modify the patient, dedicated work of many people over many decades, such as the original observers.
Your answers to these questions are earnestly sought, because there is a major problem. In the science of Metrology, there is frequent description of the need to trace measurements back to primary standards, such as the 1 metre long bar held in France for length measurements. In the branch of Meteorology that we examine, we have tried but failed to show the primary data. Therefore, emphasis has to be put on unusual patterns and events in the data being used at the present time. That is what Tom has done. A very large numbers of irregularities exist.
The data used at the present time is packaged by the BOM and sent to global centres where estimates are made of the global temperature. Tom showed at the start of this essay how the BOM has displayed and presumably endorsed a global warming pattern that has become warmer by changes made during the 21st century.
Is that displayed warming real, or an artefact of data manipulation?
That question is fundamentally important because global warming has now led to concerns of “existential crisis” and actions to stop many uses of fossil fuels. There are huge changes for all of society, so the data that leads to them have to be of high quality.
We show that it is of low quality. That is for Australia. What is known about your own countries?
It is important enough for we Australians to demand again that independent investigations, even a Royal Commission, be appointed to determine if these temperature measurements, with their serious consequences, are fit for purpose.
(END)