Adoption of AI, machine learning face challenges in healthcare
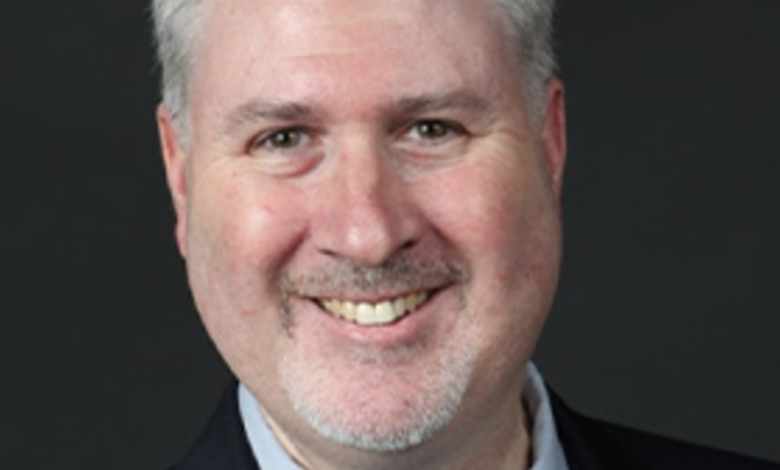
Artificial intelligence is becoming a ubiquitous part of our daily lives. It is being used to drive cars, power smart devices, create art and improve healthcare. Given the potential of AI, healthcare leaders are increasingly faced with building strong AI units and teams within their organizations.
This is no trivial task, as it requires a level of tech savvy that many leaders do not have due to its novelty and rapid development. Competent AI teams must address a wide range of critical issues such as patient safety, equity, governance, explainability, reproducibility, data drift, clinical workflows , decision support, as well as the technical details of the algorithms themselves. Let me highlight an example of the challenges healthcare leaders and their AI teams they assemble must think about if AI is going to revolutionize healthcare.
One common type of AI is machine learning, which can be used to identify patterns in electronic health record data to predict clinical outcomes. The “learning” part deals with the adaptive process of finding mathematical functions (models) that produce actionable predictions. A model is often evaluated by making predictions in new data. It is common to assess the quality of a model using measures of its predictive accuracy. While this makes sense from a mathematical point of view, it doesn’t mimic how we as humans solve problems and make decisions.
Review the car buying process. An important part of this process is deciding which car to buy. We look at make and model along with other goals like size, color, style, engine type, horsepower, range, performance, reliability and of course price. We rarely review just one feature and often don’t get everything we want. Considering multiple goals is not unique to buying a car. We go through the same process for many life decisions like choosing a college, political candidate, job, etc. These tasks are not easy, but we seem wired to make the decision. determined in this way. So why does machine learning often focus on only one goal?
One possible answer to this question is that machine learning models are often developed by AI experts who may not fully understand healthcare. Target review identifies new drug targets from machine learning models that use genetic information to predict disease risk. It is hoped that this model will show genes with protein products that can be developed into new drugs. However, for the purchase of a car, there are other important factors. For example, only about 10% of proteins have chemical properties that make them accessible to small molecule drug candidates. Information about this protein’s “drug potential” can be used to assess the value or utility of a model beyond its predictive accuracy. This goes beyond model performance to include model utility and operability.
How do we teach machine learning algorithms to choose models the same way humans buy cars? The good news is that many multi-objective methods for machine learning have been developed. They are rarely used in healthcare or other fields. An intuitive approach is known as Pareto optimization, where multiple machine learning models are created and evaluated using two or more quality criteria such as accuracy and complexity. The goal here is to define a subset of optimal equilibrium models that balance all the criteria. This approach closely mimics the car buying process.
Machine learning for healthcare is different from other application domains. Models need to do more than predict with good accuracy. They need to be transparent, unbiased, explainable, trustworthy, useful, and actionable. They need to teach us something. They need good for the patient. They need to reduce healthcare costs. This is not possible from a single objective.
An important next step with clinical AI is that computer scientists and informatics continue to work closely with clinicians to identify the right set of goals to maximize healthcare impact. health of machine learning models. This will require the involvement of the human side of the AI in addition to the algorithmic side. Healthcare leaders play an important role in assembling AI teams because they understand the required health outcome goals, they commit resources, and they can foster a culture Diversity and cooperation are necessary for success. Healthcare has its own challenges and requires an AI strategy that fits the complexity of patient care and institutional goals.