Why computers don’t need to match human intelligence
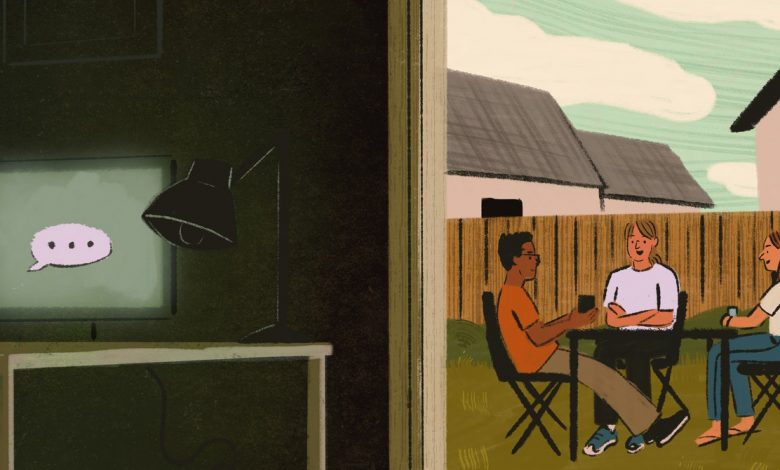
Speech and language abilities is central to human intelligence, communication and cognitive processes. Understanding natural language is often considered the best thing WHO challenge — a challenge that, if solved, could bring machines closer to human intelligence.
In 2019, Microsoft and Alibaba announced that they have built improvements to Google technology that beats humans at the natural language processing (NLP) task called reading comprehension. The news is a bit vague, but I consider it a major breakthrough because I recall what happened four years earlier.
In 2015, researchers from Microsoft and Google developed systems based on the invention of Geoff Hinton and Yann Lecun. beat humans in image recognition. At the time, I predicted that computer vision applications would bloom, and my company invested in about a dozen companies building computer vision products or applications. Today, these products are being deployed in retail, manufacturing, logistics, healthcare, and transportation. Those investments are now worth more than $20 billion.
So in 2019, when I witnessed a similar shift in human capabilities in NLP, I predicted that NLP algorithms would give rise to extreme machine translation and speech recognition capabilities. precise period, will one day power a “universal translator” as described in Star Trek. NLP will also enable entirely new applications, such as an accurate question-answering search engine (Larry Page’s grand vision for Google) and targeted content aggregation (making games today’s children’s targeted advertising). They can be used in financial, healthcare, marketing and consumer applications. Since then, we have been busy investing in NLP companies. I believe we can see a grenadepositive impact from NLP than computer vision.
What is the essence of this NLP breakthrough? It’s a technology called self-supervised learning. Previous NLP algorithms required data collection and meticulous tuning for each domain (like Amazon Alexa or a customer service chatbot for a bank), which was expensive and error-prone. But self-monitoring training works essentially all data on the world, creating a giant model that can have up to several trillion parameters.
This giant model is trained without human supervision — the AI “trains itself” by figuring out the structure of the language on its own. Then, once you have some data for a particular domain, you can fine-tune the massive model for that domain and use it for things like machine translation, question answering, and natural dialog. The fine-tuning takes parts of the giant model selectively, and it requires very little adjustment. This is a bit like how humans first learn a language and then, on that basis, learn specific knowledge or courses.
Since the 2019 breakout, we’ve seen massive NLP models grow rapidly in size (about 10x per year), with corresponding performance improvements. We’ve also seen amazing examples — such as GPT-3, which can be written in anyone’s style (such as that of Dr. Seuss), or Google Lambda, natural human-voice chat, or a Chinese startup called Langboat created Marketing materials are different for each person.
Are we going to solve the natural language problem? Skeptics claim that these algorithms are merely remembering the data of the whole world and are intelligently recalling subsets, but are clueless and not really smart. At the heart of human intelligence is the ability to reason, plan, and create.