Monitoring Arctic Ice Using Satellites, Supercomputers, and Deep Learning – Does It Work?
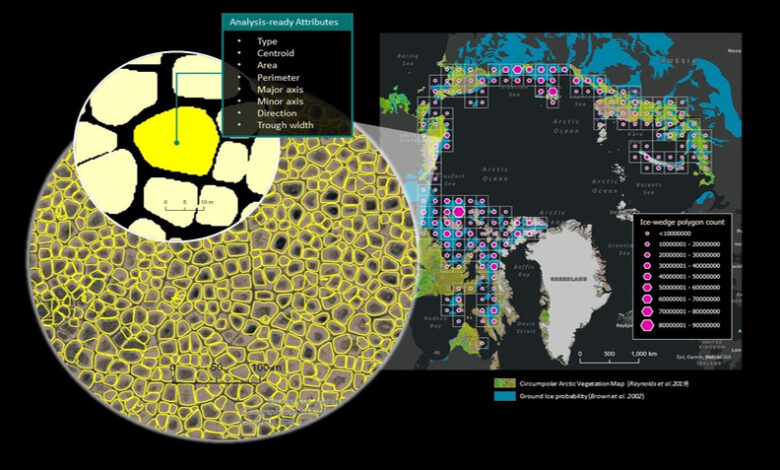
Arctic researchers and remote sensing experts use AI and HPC to characterize large unexplored parts of the Earth
UNIVERSITY OF TEXAS AT AUSTIN, TEXAS ADVANCED COMPUTER CENTER
Permafrost – ground that has been permanently frozen for two or more years – covers a large part of the Earth, about 15% of the Northern Hemisphere.
Permafrost is vital to our climate, containing large amounts of biomass stored in the form of methane and carbon dioxide, making tundra soils a carbon sink. However, the innate characteristics and changing nature of the permafrost are not widely understood.
As global warming warms the Earth and causes land to thaw, the permafrost carbon cycle is expected to accelerate and release soil-based greenhouse gases into the atmosphere, creating a feedback will exacerbate climate change.
Remote sensing is one way to deal with the breadth, dynamics, and changes to the permafrost. “It’s like a virtual passport to see this remote and hard-to-reach part of the world,” says Chandi Witharana, assistant professor of Natural Resources & Environment at the University of Connecticut. “Satellite imagery helps us to monitor the remote landscape in detail that we have never had before.”
Over the past two decades, much of the Arctic has been mapped with extreme precision by commercial satellites. These maps are a treasure trove of data about this largely unexplored region. But the data is so large and difficult to use, which makes it difficult to get scholarships, Witharana said.
With expense and support from the US National Science Foundation (NSF) as part of the “Navigate to the New Arctic” program, Witharana, as well as Kenton McHenry from the National Center for Applied Supercomputing and Arctic researchers Anna Liljedahl of the Woodwell Center for Climate Research, is making data on the Arctic permafrost much more accessible.
The team was given free access to an archive of more than 1 million photographic scenes taken in the Arctic. That’s a lot of data – so much so that traditional analysis and feature extraction methods fail. “That’s where we put AI-based deep learning methods to process and analyze this massive amount of data,” Witharana said.
One of the most striking and distinctive features of the permafrost is the ice wedge shape, which creates polygons recognizable in satellite images.
“Ice wedges form from the freezing and melting of soil in the tundra,” says Liljedahl. “Some of them are tens of thousands of years old.”
The shape and size of ice wedge polygons can provide important information about the state and rate of change in the region. But they short circuit common analyzers.
Liljedahl recalls: “I went to Facebook a few years ago and noticed that they were starting to use facial recognition software on photos. “I wonder if this applies to arctic ice wedge polygons.”
She reached out to Witharana and McHenry, whom she met during a board review in Washington, DC, and invited them to brainstorm her project. They all offer free skills in domain expertise, code development, and big data management.
Starting in 2018, Witharana started using neural networks to detect not friends’ faces but polygons from thousands of satellite images over the Arctic. To do so, Witharana and his team first had to annotate 50,000 individual polygons, hand-draw their contours, and classify them as low center or high center.
Low-centered ice wedge-shaped polygons form a pool in the center of the ridged outer part. Liljedahl says that the high center wedges look more like muffins and are evidence of the wedge melting. The two types have different hydrological structures, making it important to understand their role in climate change and future infrastructure planning in Arctic communities.
“Permafrost is not characterized at these spatial scales in climate models,” said Liljedahl. “This study will help us find a baseline and also see how changes are unfolding over time.”
Training the model with annotated images, they fed the satellite image into a neural network and tested it on unannotated data. There are early challenges – for example, images trained for Canada are less effective than in Russia, where the ice wedges are older and differently shaped. However, three years later, the team is seeing an accuracy rate of 80 to 90 percent.
They described the results of this study in ISPRS Journal of Photogrammetry and Remote Sensing (2020), Image Magazine (2020) and Explore(In 2021).
After showing that their deep learning method worked, they turned to Longhorn Supercomputeroperated by the Texas Advanced Computing Center (TACC) – a GPU-based IBM system that can perform AI inference tasks quickly – as well as Bridge-2 systems at the Pittsburgh Supercomputing Center, distributed through NSF-funded NSF Extreme Science and Engineering Exploration Environment (XSEDE)for data analysis.
As of the end of 2021, the team has identified and mapped 1.2 Billion ice wedge polygons in satellite data. They estimated that they were about half way through the full data set.
Each individual image analysis includes pre-processing (to improve image clarity and remove non-land features such as lakes), processing (where polygons are detected and characteristic) and post-processing (reducing data to a manageable rate and uploading it to a permafrost data store). In addition to identifying and classifying ice wedge polygons, this method also obtains information about the size of the wedge, the size of the trough, and other features.
Individual analyzes can be performed in less than an hour. But their sheer number makes it infeasible to run anywhere but on a mainframe, where they can be computed in parallel.
Recently, Witharana and collaborators standardized their workflow to find the optimal configuration to run efficiently on supercomputers. Writing in the journal Photogrammetric Engineering and Remote Sensing (PE&RS) in 2022, they evaluated four workflow designs on two different high-performance computing systems and found the optimal setup for speed analysis. high. A separate 2022 study in PE&RS explored the effectiveness of various image enhancement methods (such as hue or saturation changes) when applied to neural network algorithms. complex deep learning to recognize wedge-shaped polygons from commercial satellite imagery. (Both projects were presented at the American Geophysical Union Autumn meeting in December 2021.)
“Every year, we receive a near-real-time pulse oximeter in the Arctic in the form of sea ice,” says Liljedahl. “We want to do the same thing with the permafrost. There are many rapid changes. We need to be able to really understand, and communicate, what’s happening in the permafrost.”
Ice wedge data will be available for quick analysis of Permafrost . Frozen Discovery Gate, will “make information about the Arctic more accessible to more people,” Liljedahl said. “Instead of having to wait 10 years to learn about something, they can learn about it right away and discover it directly through their own experience.”
Another important phase of the research project will come when the researchers analyze satellite images representing different years and times of the year. Comparing the state of ice wedge polygons can reveal trends and trajectories, such as how quickly the landscape is changing, and where those changes will cross paths with settlements or structures. infrastructure.
“This is a perfect example of how previous investments in computing infrastructure, combined with a new understanding of deep learning techniques, are building a resource to help solve a problem. important in the Arctic,” said NSF Program Director Kendra McLauchlan.
“Man must rise above the Earth – to the top of the atmosphere and beyond – only then will he fully understand the world in which he lives,” said Plato, “Plato said. “Earth observation technologies allow us to see how climate change is happening and even how the soil is changing. It is a key tool for observing, monitoring, predicting and making decisions to prevent negative impacts on fragile areas.”
JOURNEYS
Optical Measurement & Remote Sensing Engineering
DOI
10.5194 / isprs-archives-XLIV-M-3-2021-175-2021
RESEARCH METHODS
Simulation / computational modeling
RESEARCH SUBJECTS
Do not apply
ARTICLE TITLE
Optimal GeoAI workflow for Arctic ice feature detection from high resolution satellite imagery
ARTICLE PUBLICATION DATE
January 4, 2022