Learn 3D object shapes and layouts without 3D supervision
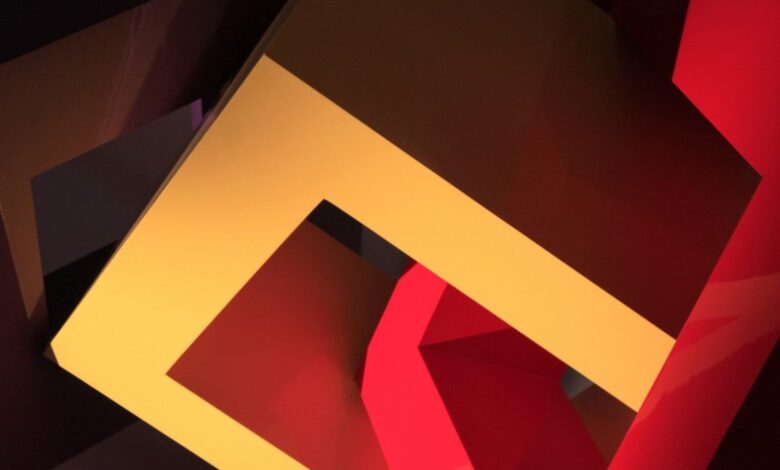
One 3D Scene can be specified with 3D shapes for each object and the 3D layout of objects in space. However, it is often impractical to measure 3D structures directly; Therefore, inferring the shape and composition of a 3D scene from a 2D image is a fundamental problem in computer vision.

An abstract 3D shape. Image credits: PxhereCC0 . public domain
A recent arXiv.org paper proposes a method for predicting 3D object shape and composition in complex scenes from a single image. It does not use realistic shapes or compositions during training, and object shadows in the multi-view image are used for learning.
Mesh R-CNN, 3D shape prediction, enhanced with a layout network that estimates the 3D position of each object. The results on three data sets show the utility of scalable multi-view monitoring. The approach scales to complex, realistic scenes with a wide range of subjects and can learn from noisy real-world video without expensive truths.
A 3D scene consists of a set of objects, each with a shape and layout that indicates their position in space. Understanding 3D scenes from 2D images is an important goal, with applications in robotics and graphics. Although there have been recent advances in 3D shape and composition prediction from a single image, most methods rely on ground-based 3D truths to train, which is expensive to train. collected on a large scale. We overcome these limitations and propose a learning method that predicts the 3D shape and layout of objects without any realistic shape or composition information: instead, we rely into multi-mode images with 2D surveillance that can be easily collected on a larger scale. Through extensive tests on 3D Warehouse, Hypersim and ScanNet, we demonstrate that our approach extends to large datasets of real-life images and compares favorably with methods based on on 3D ground truth. On Hypersim and ScanNet, where reliable ground 3D facts are not available, our approach outperforms supervised approaches trained on smaller and less diverse datasets .
Research articles: Gkioxari, G., Ravi, N. and Johnson, J., “Learning about 3D object composition and shape without 3D supervision”, 2022Link: https://arxiv.org/abs/2206.67028