Guardrails, data governance key to solid generative AI outcomes
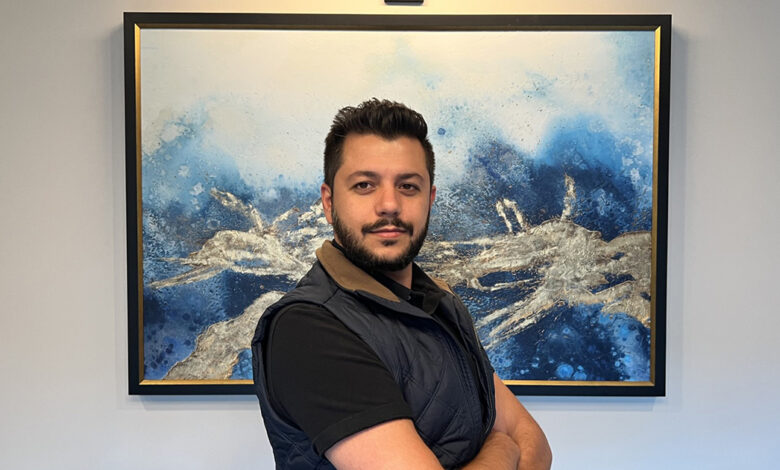
There is growing concern among some health tech leaders that they should perhaps take a step back to ensure the use of artificial intelligence – especially generative AI – is safe, appropriate, secure and morally sound.
Its potential benefits are huge when used in conjunction with human guidance, leading to early diagnosis, improved disease prevention and overall wellness with the correctly “tuned” prediction algorithms. But the alarm has been sounded by some about AI usage already leading to more of a digital divide, creating further bias, and driving inequity.
Gharib Gharibi, who holds a PhD in computer science, is director of applied research and head of AI and privacy at TripleBlind, an AI privacy technology company. He has strong opinions – based on his own research and experience training large language models – that AI should be seen as augmented intelligence, successful only with human interaction and support. Healthcare IT News spoke with him to get his perspective on this and other topics.
Q. You say there is a growing digital divide and biases stemming from the misuse of generative AI in healthcare today. Please explain.
A. Generative AI, and AI algorithms in general, are programs that generalize from data; and if the data used already is biased, the AI algorithm will be, too.
For example, if a generative model is trained on medical images collected from a single source, located in a geographical area with predominant ethnic population, the trained algorithm will most likely fail to operate accurately for other ethnic groups (assuming patient’s ethnicity is a good predictive variable).
Generative AI in particular has the ability to create synthetic patient data, simulate disease progress, and even generate realistic medical images for training other AI systems. Using single-source, biased data to train such systems can, therefore, mislead academic research, misdiagnose diseases and generate ineffective treatment plans.
However, while diversifying data sources, for both training and validation, can help minimize bias and generate more accurate models, we should pay close attention to patients’ privacy. Sharing healthcare data can raise significant privacy concerns, and there’s an immediate and significant need to strike the right balance between facilitating data sharing and protecting patients’ privacy.
Finally, there’s the ongoing debate about regulating AI in healthcare to reduce intentional and unintentional misuse of this technology. Some regulation is necessary to protect patients’ safety and privacy, but we have to be careful with that, too, because too much regulation will hamper innovation and slow down the creation and adoption of more affordable, lifesaving AI-based technologies.
Q. Please talk about your research and experience training large language models and from that your opinion that AI should be seen as augmented intelligence, successful only with human interaction and support.
A. My experience and research interests fall at the intersections of AI, systems and privacy. I am passionate about creating AI systems that can facilitate human lives and augment our tasks accurately and efficiently while protecting some of our fundamental rights – security and privacy.
Today, AI models themselves are designed to work in tandem with human users. While AI systems, such as ChatGPT, can generate responses to a wide range of prompts, they still rely on humans to provide these prompts. It still does not have goals or “desires” of their own.
Its main goal today is to assist users in achieving their objectives. This is particularly relevant in the healthcare domain, where the ability to process sensitive data quickly, privately and accurately can improve diagnosis and treatments.
However, despite generative AI models’ powerful abilities, they still generate inaccurate, inappropriate and biased responses. It could even leak important information about the training data, violating privacy; or be easily fooled by adversarial input examples to generate wrong results. Therefore, human involvement and supervision is still critical.
Looking ahead, we will witness the emergence of fully automated AI systems, capable of tackling extensive, intricate tasks with no need for human intervention. These sophisticated generative AI models could be assigned complex tasks, such as predicting all potential personalized treatment plans and outcomes for a cancer patient.
It would then be able to generate comprehensive solutions that would otherwise be impossible for human experts to achieve.
The immense data handling capabilities of AI systems, far exceeding the cognitive limits of human beings, are crucial in this context. Such tasks also demand computations that would take a human lifetime or more to complete, making them impractical for human experts.
Finally, these AI systems are not subject to fatigue and do not get sick (albeit they face other types of issues, such as concept drift, bias, privacy, etc.), and they can work relentlessly around the clock, providing consistent results. This aspect alone could revolutionize industries where constant analysis and research is crucial, such as healthcare.
Q. What are some of the guardrails you believe should be put in place with regard to generative AI in healthcare?
A. As we move toward a future where generative AI becomes more integrated into healthcare, it’s essential to have robust guardrails in place to ensure the responsible and ethical use of these technologies. Here are a few key areas where safeguards should be considered:
1. Data privacy and security. AI in healthcare often involves sensitive patient data, and therefore robust data privacy and security measures are crucial. This includes using and improving current privacy-enhancing methods and tools like blind learning, secure multiparty computation (SMPC), federated learning and others.
2. Transparency. It’s important for healthcare providers and patients to understand how AI models make predictions. This could involve providing clear explanations of how the AI works, its limitations, and the data it was trained on.
3. Bias mitigation. Measures should be in place to prevent and correct biases in AI. This involves diverse and representative data collection, bias detection and mitigation techniques during model training, and ongoing monitoring for bias in AI predictions.
5. Regulation and accountability. There should be clear regulations governing the use of AI in healthcare, and clear accountability when AI systems make errors or cause harm. This may involve updating existing medical regulations to account for AI, and creating new standards and certifications for AI systems in healthcare.
6. Equitable access. As AI becomes an increasingly important tool in healthcare, it’s crucial to ensure that access to AI-enhanced care is equitable and doesn’t exacerbate existing health disparities. This might involve policies to support the use of AI in underserved areas or among underserved populations.
Setting up these guardrails will require collaboration among AI scientists, healthcare providers, regulators, ethicists and patients. It’s a complex task, but a necessary one to ensure the safe and beneficial use of generative AI in healthcare.
Q. What are some of the data management techniques you believe will help providers avoid biased results?
A. Reducing bias in privacy-preserving, explainable AI systems requires careful and effective data management, design and evaluation of the complete AI system pipeline. In addition to what I already mentioned, here are several techniques that can help healthcare providers avoid biased results:
1. Diverse data collection. The first step to avoiding bias is ensuring that the data collected is representative of the diverse populations the AI will serve. This includes data from individuals of different ages, races, genders, socioeconomic statuses and health conditions.
2. Data preprocessing and cleaning. Prior to training an AI model, data should be preprocessed and cleaned to identify and correct any potential sources of bias. For instance, if certain groups are underrepresented in the data, techniques like oversampling from these groups or undersampling from overrepresented groups can help to balance the data.
3. Bias auditing. Regular audits can help identify and correct bias in both the data and the AI models. This involves reviewing the data collection process, examining the data for potential biases, and testing the AI model’s outputs for fairness across different demographic groups.
4. Feature selection. When training an AI model, it’s important to consider which features or variables the model is using to make its predictions. If a model is relying heavily on a feature that is biased or irrelevant, it may need to be adjusted or removed.
5. Transparent and explainable AI. Using AI models that provide clear explanations for their predictions can help identify when a model is relying on biased information. If a model can explain why it made a certain prediction, it’s easier to spot when it’s basing its decisions on biased or irrelevant factors.
Ultimately, managing bias in AI requires a combination of technical solutions and human judgment. It’s an ongoing process that requires continuous monitoring and adjustment. And it’s a task that is well worth the effort, as reducing bias is essential for building AI systems that are fair, reliable and beneficial for all.
Follow Bill’s HIT coverage on LinkedIn: Bill Siwicki
Email him: [email protected]
Healthcare IT News is a HIMSS Media publication.