From one hand to many hands: Learn to imitate the skillful maneuvers from the remote control via the single camera
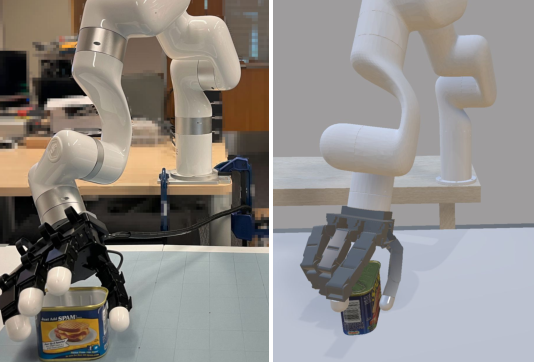
Skillful Manipulation with multi-fingered hands is a difficult problem due to the complex contact pattern between the hand and the objects being manipulated. One of the solutions is to learn from a human demonstration collected by remote control.

Downside: real robot setup. The cyan poster on the table is a reference coordinate to determine if the object is moved to the target location. Right edge: simulated robotic arm setup. Image credit: Yuzhe Qin, Hao Su, Xiaolong Wang
A recent article published on arXiv.org introduces a single-camera remote control system with an expandable setup and intuitive control interface that can collect demonstrations for multiple roboticists.
The system uses the iPad request as the capture device. First, an operator’s hand geometry is estimated. The system generates a custom robotic arm that resembles the similar kinematics of the operator’s hand.
Once the demonstrations are collected by the custom robot hand, the trajectory of the custom robot arm will be converted to the actual designated robot arm. Experiments with dexterity tasks such as Reposition, Flip and Door Open show great advantages over previous methods.
We propose to implement mimetic learning to skillfully manipulate multi-finger robot hands from human demonstrations and transfer policy to real robot hands. We introduce a new single-camera remote control system to efficiently collect 3D illustrations with only iPads and computers. An important contribution of our system is that we create a custom robotic hand for each user in the physics simulator, which is a manipulator that resembles the kinematics and geometry of the robot. operator’s hand. This provides an intuitive interface and avoids unstable human-robot manual retargeting for data collection, resulting in high-quality and large-scale data. Once the data is collected, the custom robotic arm trajectories can be converted to different specified robotic arms (manufactured models) to generate training demonstrations. With mimetic learning using our data, we show great improvement over baselines with many complex manipulative tasks. More importantly, we show that our learned policy is significantly stronger when moving to real robots. More videos can be found in This https URL .
Research articles: Qin, Yuzhe et al. “From one hand to many hands: Learning to imitate to skillfully manipulate from a single camera remote control”, 2022. Link: https://arxiv.org/abs/2204.12490