Controversy surrounding the Sun’s role in climate change
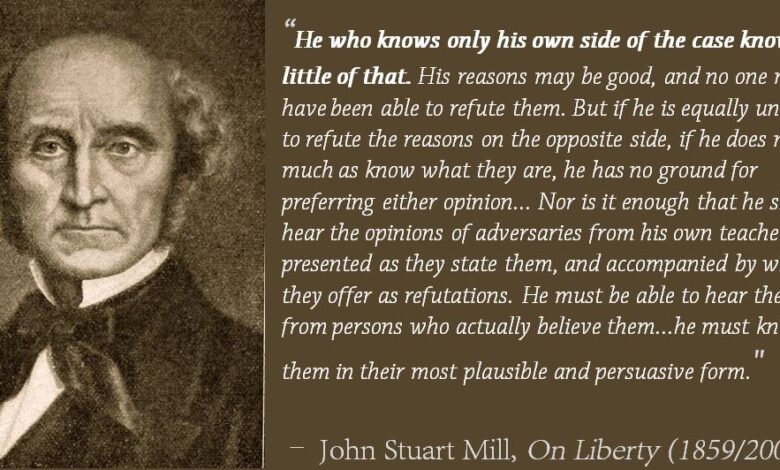
by Dr. Willie Soon, Dr. Ronan Connolly & Dr. Michael Connolly
Gavin Schmidt at realclimate.org attempts to dismiss our recent papers, including pseudo-scientific takedowns. This post takes a deep dive into the controversies.
In the last month, we have co-authored three papers in scientific peer-reviewed journals collectively dealing with the twin problems of (1) urbanization bias and (2) the ongoing debates over Total Solar Irradiance (TSI) datasets:
- Soon et al. (2023). Climate. https://doi.org/10.3390/cli11090179. (Open access)
- Connolly et al. (2023). Research in Astronomy and Astrophysics. https://doi.org/10.1088/1674-4527/acf18e. (Still in press, but pre-print available here)
- Katata, Connolly and O’Neill (2023). Journal of Applied Meteorology and Climatology. https://doi.org/10.1175/JAMC-D-22-0122.1. (Open access)
All three papers have implications for the scientifically challenging problem of the detection and attribution (D&A) of climate change. Many of our insights were overlooked by the UN’s Intergovernmental Panel on Climate Change (IPCC) in their last three Assessment Reports (AR), i.e., IPCC AR4 (2007), IPCC AR5 (2013) and IPCC AR6 (2021). This means that the IPCC’s highly influential claims in those reports that the long-term global warming since the 19th century was “mostly human-caused” and predominantly due to greenhouse gas emissions were scientifically premature and the scientific community will need to revisit them.
So far, the feedback on these papers has been very encouraging. In particular, Soon et al. (2023) seems to be generating considerable interest, with the article being viewed more than 20,000 times on the journal website in the first 10 days since it was published.
However, some scientists who have been actively promoting the IPCC’s attribution statements over the years appear to be quite upset by the interest in our new scientific papers.
This week (September 6th, 2023), a website called RealClimate.org published a blog post by one of their contributors, Dr. Gavin Schmidt, the director of the NASA Goddard Institute for Space Studies (NASA GISS). In this post, Dr. Schmidt is trying to discredit our analysis in Soon et al. (2023), one of our three new papers, using “straw-man” arguments and demonstrably false claims.
As we summarize in Connolly et al. (2023),
“A “straw man” argument is a logical fallacy where someone sets up and then disputes a position that was not actually made by the group being criticised. Instead, the group’s arguments or points are either exaggerated, misrepresented, or completely fabricated by the critics.”
In our opinion, while this rhetorical technique might be good for marketing, political campaigning, “hit pieces”, etc., it is not helpful for either science or developing informed opinions. Instead, we strive in our communications to take a “steel-manning” approach. As we point out in Connolly et al. (2023),
“Essentially, this involves addressing the best and most constructive form of someone’s argument – even if it is not the form they originally presented.”
With that in mind, we will first steel-man Dr. Schmidt’s apparent criticisms of Soon et al. (2023).
Steel-manning Dr. Schmidt’s criticisms of Soon et al. (2023)
In his latest RealClimate post, Dr. Schmidt claims the following:
- He asserts that one of the two Total Solar Irradiance (TSI) time series that we considered in Soon et al. (2023) is flawed, out-dated and unreliable. (As an aside, this was also one of the 27 TSI series we considered in Connolly et al. (2023) but he does not discuss this paper here)
- He claims that a 2005 paper by Dr. Willie Soon looking at the relationship between TSI and Arctic temperatures has been disproven by the passage of time.
- He argues that the “rural-only” Northern Hemisphere land surface temperature record that was one of the two temperature records we analysed in Soon et al. (2023) is not representative of rural global temperature trends or even rural Northern Hemisphere temperature trends.
Later in this post, we will respond to each of Dr. Schmidt’s claims and show how they are incorrect. But, first it may be useful to provide some background information on RealClimate.org.
How reliable is RealClimate.org?
RealClimate.org was created in 2004 as a blog to promote the scientific opinions of the website owners. It is currently run by five scientists: Dr. Gavin Schmidt, Prof. Michael Mann, Dr. Rasmus Benestad, Prof. Stefan Rahmstorf and Prof. Eric Steig.
Anybody with scientific training (or even just a careful reader) who actually reads our paper will be able to see that each of the claims in Dr. Schmidt’s recent blog-post are either false, misleading or already clearly addressed by our paper. Therefore, scientifically speaking, his post doesn’t contribute in any productive or meaningful way.
Instead, unfortunately, the goal of his post seems to be to try and stop inquiring minds from reading our paper.
If people were only to read his blog-post then they might be discouraged from even looking at our paper – and therefore wouldn’t find out that Dr. Schmidt’s alleged “criticisms” are without merit.
This type of pseudoscientific “take-down” of any studies that disagree with the RealClimate team’s scientific opinions seems to be a common pattern. For example, in November, they posted a similar “take-down” of our 2021 study that they disdainfully titled “Serious mistakes found in recent paper by Connolly et al.” That post summarized their attempted “rebuttal” of our Connolly et al. (2021) paper by Richardson & Benestad (2022).
Anybody who reads both Connolly et al. (2021) and Richardson & Benestad (2022) will quickly realize that their attempted “rebuttal” was also easily disproven. Indeed, two of our three recent papers explicitly demonstrate that Richardson & Benestad (2022)’s claims were flawed and erroneous.
Again, it seems that the goal of Richardson & Benestad (2022) and RealClimate’s accompanying post in November was NOT to further the science, but rather to discourage people from actually reading Connolly et al. (2021)!
Connolly et al. (2023) is our formal reply to Richardson & Benestad (2022)’s attempted rebuttal of our earlier Connolly et al. (2021) paper.
For anybody who is wondering what our response to Richardson & Benestad’s November 2022 RealClimate post is, we recommend reading the full papers themselves.
All three articles were published in the peer-reviewed journal, Research in Astronomy and Astrophysics (RAA for short).
- Connolly et al. (2021): here
- Richardson & Benestad (2022): here
- Our reply, Connolly et al. (2023): abstract; preprint here (final version is still being typeset)
Addressing claim 1: What is the most reliable TSI time series available?
A key challenge that is the subject of considerable ongoing scientific debate and controversy is the question of how Total Solar Irradiance (TSI) has changed since the 19th century and earlier.
It was only in late 1978, during the satellite era, that it became possible to directly measure the TSI from above the Earth’s atmosphere using TSI-measuring instruments onboard satellites.
Even during the satellite era, it is still unclear exactly how TSI has changed because:
- Each satellite mission typically only remains active for 10-15 years.
- Each satellite instrument gives a different average TSI value.
- Each satellite implies subtly different, but significant differences in the trends between each sunspot cycle.
These problems can be seen below:
We can see from the above that, even though the data from each satellite mission are different, all of the instruments record the increases and decreases in solar activity over the roughly 11 year “solar cycle” which is observed in many solar activity indicators.
However, because the individual instruments typically only cover 10-15 years and they show different underlying trends relative to each other, it is unclear what other trends in TSI have occurred over the satellite era.
Several different research teams have developed their own satellite composites by combining the above satellite data in different ways and using different assumptions and methodologies. See this CERES-Science post for a summary.
Some of the main recent composites are:
- “ACRIM” – The ACRIM group finds that in addition to the 11 year solar cycle, there are important trends between each cycle. They find there was a significant increase in TSI between each solar minimum and maximum in the 1980s and 1990s, followed by a slight decrease in the early 2000s. See e.g., Scafetta et al. (2019).
- “PMOD” – The PMOD group applies several adjustments to the data of some of the early satellite missions and uses different methodological choices and assumptions. They find there has been a slight decrease in TSI between each of the cycles, but that it has been quite modest. See e.g., Montillet et al. (2022).
- “RMIB/ROB” – The ROB group (previously called RMIB) argues that there has been almost no change in TSI over the satellite era other than the 11 year solar cycle. See e.g., Dewitte & Nevens (2016).
- “The Community Composites” – Dudok de Wit et al. (2017) offer two different TSI composites. One using the original satellite data implies a reconstruction intermediate between the RMIB and ACRIM composites. The other using the PMOD-adjusted satellite data implies a reconstruction similar to the PMOD composite.
For the pre-satellite era, we don’t have direct measurements. Instead, researchers have to rely on “solar proxies” that they hope are accurately capturing important aspects of changing solar activity.
Some of the proxies include: sunspot numbers, group sunspot numbers, solar cycle lengths, the ratios of penumbra and umbra features of sunspots, bright spots in the sun’s photosphere (called solar faculae), cosmogenic isotope measurements, etc.
Typically, the solar proxies are calibrated against the satellite measurements during the satellite era. The calibrated solar proxies are then used to estimate the changes in TSI during the pre-satellite era.
Already you are probably thinking this is a complex and challenging problem. You are correct! Although, some scientists act as if these problems have all been fully resolved, those of us who have been actively researching these problems for many years can tell you that it is a thorny and contentious subject.
Depending on (a) which satellite composite is used; (b) which solar proxies are used; and (c) what methodologies are used, different research teams can develop very different long-term TSI reconstructions.
For example,
- Matthes et al. (2017) is the one IPCC AR6 used – based on the average of two other TSI reconstructions – Krivova et al. (2007)’s “SATIRE” and Coddington et al. (2016)’s “NRLTSI2”. All three match well to the PMOD composite.
- Dewitte et al. (2022) is a simple reconstruction based on simply rescaling the sunspot number record to match the RMIB composite.
- Egorova et al. (2018) developed 4 different estimates. Each shows far more variability over the last few centuries than the IPCC estimates.
- In a 2019 NASA study, Scafetta et al. (2019) updated the original Hoyt and Schatten (1993) TSI reconstruction using the ACRIM composite. This is a somewhat unique reconstruction because unlike most of the other reconstructions that only use one or two solar proxies, Hoyt and Schatten used five solar proxies in order to capture multiple aspects of solar variability.
- Penza et al. (2022) implies that TSI has changed significantly over the past century or so, but not as much as the Egorova et al. (2018) or the updated Hoyt and Schatten (1993) reconstruction.
In Connolly et al. (2023), we analysed a total of 27 TSI reconstructions, including all of the above. However, in Soon et al. (2023), for simplicity, we focused on just two of the above reconstructions – Matthes et al. (2017) and the ACRIM-updated Hoyt & Schatten reconstruction.
Dr. Schmidt clearly does not like either the ACRIM composite or Hoyt & Schatten’s original TSI composite. We can understand why! It implies a much larger role for the Sun in the climate changes since the 19th century than the RealClimate team claims exist.
However, it is worth noting that Hoyt and Schatten (1993) was used as one of the 6 TSI series considered by the CMIP3 modelling project that the IPCC used for their detection and attribution analysis in their 4th Assessment Report (2007). This can be confirmed by checking the list of “SOL” (i.e., TSI) time series on pages 11-12 of the Supplementary Material for Chapter 9 of IPCC AR4 Working Group 1.
The CMIP5 and CMIP6 modelling projects that contributed to the 2013 and 2021 AR5 and AR6 reports did not consider Hoyt and Schatten (1993) as a potential TSI series. However, this seems to partly be due the influence of Dr. Schmidt. He was the lead author of Schmidt et al. (2011), i.e., the paper recommending the climate modelling forcings to be used for the PMIP3 and CMIP5 projects.
At any rate, as we mentioned above, in Connolly et al. (2023) we considered a total of 27 different TSI reconstructions and we still reached similar conclusions to Soon et al. (2023). So, the specific choice of Hoyt and Schatten is just one way to demonstrate that the IPCC was premature in their AR6 attribution.
Addressing claim 2: Was Soon (2005) wrong?
In Soon (2005), Dr. Soon noticed a remarkable correlation between the Hoyt and Schatten (1993) TSI series and Arctic temperatures from 1875 to 2000.
In a follow-up paper, Soon (2009) repeated his analysis using (a) a newer version of the Hoyt and Schatten TSI series that had been updated to 2007 and (b) NASA GISS’ Arctic temperature record from 1880-2007.
The comparison is shown below adapted from Figure A.1 of Soon (2009):
In this week’s blog post, Dr. Schmidt conceded that the fit looked ok in 2005, but he claims it no longer holds:
“But time marches on, and what might have looked ok in 2005 (using data that only went to 2000) wasn’t looking so great in 2015.”
Dr. Schmidt then showed a plot he did in 2015 using a different TSI record from that used by Soon. His reanalysis failed to identify a compelling correlation when applied to the updated NASA GISS Arctic temperature record.
On the other hand, also in 2015, as part of our analysis of Northern Hemisphere rural temperature trends, in Soon et al. (2015) we included our own update to the original Soon (2005; 2009) analysis.
Below is Figure 27(d) of Soon et al. (2015). The blue line represents Arctic temperatures, while the dashed red line represents TSI:
Dr. Schmidt’s attempt to update Soon (2005)’s analysis to 2015, by using a different TSI record, failed.
In contrast, Soon et al. (2015)’s update’s that used the updated version of the TSI used by Soon (2005) and Soon (2009) confirmed the original findings of the earlier studies.
Addressing claim 3: Is our rural-only Northern Hemisphere temperature record representative of genuine climate change?
Dr. Schmidt appears to be confused about the rural-only Northern Hemisphere temperature record that we used as one of two comparative temperature records in Soon et al. (2023) and also as one of five comparative temperature records in Connolly et al. (2023).
We are surprised that he does not seem to have understood how these temperature records were constructed, since the construction of all five temperature records was described in detail in Connolly et al. (2021), along with a detailed discussion of the rationale for each temperature record. Details were also provided in the Soon et al. (2023) paper he was criticizing.
However, perhaps he hasn’t actually taken the time to read Connolly et al. (2021) yet. When Connolly et al. (2021) was published, Dr. Schmidt was asked to comment on the paper by a journalist for The Epoch Times. According to The Epoch Times:
“When contacted about the new paper, Gavin Schmidt, who serves as acting senior advisor on climate at NASA and the director of the Goddard Institute for Space Studies, was also blunt.
“This is total nonsense that no one sensible should waste any time on,” he told The Epoch Times.
He did not respond to a follow-up request for specific errors of fact or reasoning in the new RAA paper.”
– The Epoch Times, August 16th, 2021
One of the key problems we were highlighting in Soon et al. (2023) was the so-called “urbanization bias problem”. It is well-known that urban areas are warmer than the surrounding countryside. This is known as the “Urban Heat Island (UHI)” effect.
Because urban areas still only represent 3-4% of the global land surface, this should not substantially influence global temperatures.
However, most of the weather stations used for calculating the land component of global temperatures are located in urban or semi-urban areas. This is especially so for the stations with the longest temperature records. One reason why is because it is harder to staff and maintain a weather station in an isolated, rural location for a century or longer.
As a result, many of the longest station records used for calculating global temperature changes have probably also experienced localized urban warming over the course of their records. This urban warming is not representative of the climate changes experienced by the non-urban world.
Urban warming that gets mistakenly incorporated into the “global temperature” data is referred to as “urbanization bias”.
It is still unclear exactly how much the current global warming estimates are contaminated by urbanization bias. In their most recent report, the IPCC stated optimistically that urbanization bias probably accounted for less than 10% of the land warming. However, they did not offer a robust explanation for why they felt this was so.
Indeed, as described in Connolly et al. (2021), Soon et al. (2023) and Connolly et al. (2023), several scientific studies have suggested that urban biases accounted for more than 10% of the land warming – and possibly much more.
Brief detour: background to how and why we developed our rural-only Northern Hemisphere temperature record
In Connolly et al. (2021), we attempted to resolve the urbanization bias problem by developing a rural-only temperature record that only used temperature records from rural stations or stations that had been explicitly corrected for urbanization bias. However, we faced two major problems that we had been trying to resolve for nearly a decade:
- There was a severe shortage of rural stations with temperature records of a century or longer.
- Many weather stations with long temperature records are contaminated by other non-climatic biases, such as station moves, changes in instrumentation, etc.
When we looked at how other international groups (including Dr. Schmidt’s group at NASA GISS) were accounting for the non-climatic biases in the data, we discovered that they were not making any effort to contact the station owners for “station history metadata”, i.e., information on any changes associated with the station over its record.
Instead, most groups (including NASA GISS), were relying on automated computer programs that tried to guess when station changes might have introduced a bias. These programs used statistical algorithms that compared each station record to those of neighboring stations and applying “homogenization adjustments” to the data.
In a series of three “working papers”, two of us (Dr. Ronan Connolly and Dr. Michael Connolly) had published in 2014, we described how:
- There were serious flaws in the homogenization algorithms used by NOAA, the group whose homogenized data NASA GISS used for their global temperature estimates. (https://oprj.net/articles/climate-science/34)
- There were also serious problems with the additional “urbanization bias” adjustment computer program that NASA GISS applied to NOAA’s homogenized data (see https://oprj.net/articles/climate-science/31)
- All of the published studies up until at least 2013 that purported to have ruled out urbanization bias as a substantial problem had methodological flaws that meant their conclusions were invalid (see http://oprj.net/articles/climate-science/28)
In April 2015, while we were visiting New York City (along with Dr. Imelda Connolly), we offered to discuss these problems with Dr. Schmidt at the NASA GISS offices and see if possible solutions could be found. Dr. Schmidt declined the invitation explaining that he was not very familiar with how NASA GISS’ global temperature dataset was constructed. But, he kindly arranged for us to discuss the working papers with Dr. Reto Ruedy who was the lead scientist in charge of NASA GISS’ temperature dataset (called “GISTEMP”) at the time. All four of us (Dr. Reto Ruedy, Dr. Imelda Connolly, Dr. Michael Connolly and Dr. Ronan Connolly) met in the iconic “Tom’s Restaurant” right beside the NASA GISS office building.
Dr. Ruedy admitted that none of their research team had considered the various problems we had raised in our working papers. We asked him if he could see any problems with our analysis. He said that he couldn’t immediately, but he promised to e-mail us if he could see any mistakes in our analysis. Since he never e-mailed us, we assume that he couldn’t find any errors.
During our meeting with Dr. Ruedy, we warned him of a problem with NOAA’s homogenization algorithm which we call the “urban blending” problem, but others have called the “statistical aliasing problem”. Recently we have confirmed the severity of this problem in Katata et al. (2023).
We warned him that because of this problem, the urban warming biases in the data would become more deeply embedded in his global temperature estimates if he used NOAA’s homogenized version of the data.
He admitted that this was a problem, but he explained that the NASA GISS team in charge of the GISTEMP dataset were only allocated a limited number of hours per week to work on the data. So, he said that they had to trust that NOAA’s homogenization efforts were better than nothing.
We left our meeting with Dr. Ruedy quite disappointed to discover that even NASA GISS’ well-resourced research team didn’t know how to resolve these key scientific problems and were effectively just hoping that someone else was looking after the data.
We decided, if nobody else was going to try and properly resolve these problems, we would try.
At the time, NOAA provided two types of urban ratings associated with each of their Global Historical Climatology Network (GHCN) dataset – one based on local populations and the other based on the intensity of night-lights in the area. This was version 3 of the GHCN and NOAA kept it updated until late 2019. The current version of GHCN (version 4) does not include any urbanization metrics. So, for now our main analysis has focused on version 3. However, we are working on expanding our analysis in the future using version 4 – see Soon et al. (2018); O’Neill et al. (2022) and Katata et al. (2023) for more details on this later work.
We decided to sort all 7200 of the GHCN stations into three categories – “rural” stations are those rural in terms of both GHCN urban metrics; “urban” stations are those urban in terms of both metrics. All other stations we classify as “semi-urban”.
Immediately, we found several problems:
- Less than 25% of the GHCN stations are “rural”.
- Most of the rural stations had very short station records – often only covering 40-50 years or so.
- The few rural stations that had long records reaching back to the late-19th century or early-20th century were almost entirely in the Northern Hemisphere – and confined to a few regions: North America, Europe, East Asia and several Arctic locations.
- Many of the rural stations with nominally long station records often contained large data gaps and sudden shifts in the average temperature that could potentially be due to non-climatic station changes.
Most of the groups generating global temperature records from the weather station data rely on the temperature homogenization computer programs mentioned above to automatically adjust the original temperature records to remove “non-climatic biases” from the data.
If these homogenization computer programs were as reliable as many scientists have assumed, then these “automatically homogenized” temperature records should no longer be contaminated by non-climatic biases.
However, as we had discovered (and discussed with Dr. Ruedy in our 2015 meeting), these homogenization algorithms have serious statistical problems that introduce new non-climatic biases into the homogenized data.
In subsequent years, we have demonstrated the severity of these biases and statistical problems in several peer-reviewed scientific articles: Soon et al. (2018); O’Neill et al. (2022) and the recent Katata et al. (2023).
Therefore, we realised that the other groups analysing the weather station data were inadvertently making a major scientific blunder by relying uncritically on this automatically “homogenized” data.
Instead, to correct for non-climatic biases in the data, we need to start making more realistic experimentally-based corrections.
We decided to begin our analysis by identifying the areas with the longest rural records and the most information on the non-climatic biases associated with the data. We found that just four regions accounted for more than 80% of all the rural stations with data for the late-19th century/early-20th century. All four regions are in the Northern Hemisphere.
In our opinion, there was simply not enough Southern Hemisphere rural data to construct a global rural-only temperature series that would reach back to the late-19th century.
Therefore, we confined our analysis to the more data-rich Northern Hemisphere.
One region where we think we can expand our analysis in the future is for Europe (as we discuss in the papers). Currently, our European rural temperature analysis is confined to Ireland because we were able to obtain the key station history metadata for the Irish stations from the national meteorological organization (Met Éireann). However, in a recent paper, O’Neill et al. (2022), we carried out a large collaborative effort with scientists across Europe to compile the station history metadata for more than 800 weather stations in 24 European countries – see here for a summary. Most of these European stations are urbanized, but our preliminary analysis suggests that we should be able to use this metadata in the future to develop a more data-rich “rural Europe” temperature record.
How does our rural-only series compare to the standard “urban and rural” record?
The top panel shows the standard Northern Hemisphere land temperature estimates using all stations – urban as well as rural. The bottom panel shows our rural-only temperature estimate.
In Soon et al. (2023) – the paper Dr. Schmidt was complaining about – we consider both temperature estimates. We also consider two different TSI records – the Matthes et al. (2017) TSI series used for the attribution experiments in IPCC AR6 and the updated Hoyt and Schatten TSI series that we discussed earlier.
See below for a summary of some of our key findings:
Dr. Schmidt complains that our Northern Hemisphere rural-only temperature record is not reliable because,
“It’s not a good areal sample of the northern hemisphere, it’s not a good sample of rural stations – many of which exist in the rest of Europe, Australia, Southern Africa, South America etc., it’s not a good sample of long stations (again many of which exist elsewhere).”
This is a rather clever, but deceptive, misrepresentation of the data. Notice how he splits up his statement into two parts.
For the first part, he correctly says that there is “a good sample of rural stations” in other regions from those we analysed, but he neglects to explain that they are mostly stations with short records.
Indeed, if we look at the number of stations available in the year 2000, there are indeed many rural stations around the globe:
Then in the second part of his statement, he correctly says that there are many long station records outside of the regions we analysed, but he neglects to explain that they are not rural!
Below are the stations with data available in 1880. We have highlighted the three Southern Hemisphere regions he implied we should have also incorporated, i.e., Australia, Southern Africa and South America:
Do you see why Dr. Schmidt’s characterization of the available data was disingenuous?
But, what about other estimates of Northern Hemisphere temperatures?
In Soon et al. (2023), we were assessing the Northern Hemisphere land surface warming (1850-2018) based on the weather station data. However, in Connolly et al. (2023), we also considered an additional three Northern Hemisphere temperature series. One was generated from the Sea Surface Temperature (SST) data. The other two were based on temperature proxy data: (a) tree-ring temperature proxies or (b) glacier-length temperature proxies.
Dr. Schmidt claimed that our rural-only temperature series was not representative of Northern Hemisphere temperature trends. If he is correct, then presumably these other temperature estimates would show very different trends. Let’s see!
What do you think?
Obviously, they are not exactly identical. However, in our opinion, all three of these alternative temperature estimates are broadly similar to our rural-only temperature record.
Therefore, we disagree with Dr. Schmidt’s claim.
Finally, if you recall from the beginning, Dr. Schmidt didn’t like the second TSI series we analysed in Soon et al. (2023). However, in Connolly et al. (2023), we analysed a total of 27 TSI series.
For each temperature record, we carried out statistical analysis in terms of the “natural” and “anthropogenic” (i.e., human-caused) climate drivers that the IPCC used for their attribution experiments. The IPCC only considered two natural drivers – TSI and volcanic eruptions. We used all of the IPCC’s climate driver records, but we repeated our analysis using each of the 27 TSI series in turn.
In both Connolly et al. (2023) and Soon et al. (2023), we adopt a similar approach to the IPCC’s attribution analysis. That is, we compare the results you get by considering:
- “Only natural factors” (which the IPCC defines as TSI and volcanic)
- “Only anthropogenic factors”.
- “Natural and anthropogenic factors”
If you want to see the results from all of these combinations, we recommend reading the full papers. But, for simplicity, let us just compare the first two combinations.
In Figure 6 of Connolly et al. (2023), we compared the “natural factors only” fittings using three of the best-fitting TSI series to the “anthropogenic factors only” fits. See below:
The TSI record that Dr. Schmidt was complaining about is labelled here as “H1993”. But, notice how for each temperature record, we can obtain similarly good fits using other TSI estimates.
Conclusions
In Soon et al. (2023), we reached the following key conclusions:
“(1) urbanization bias remains a substantial problem for the global land temperature data; (2) it is still unclear which (if any) of the many TSI time series in the literature are accurate estimates of past TSI; (3) the scientific community is not yet in a position to confidently establish whether the warming since 1850 is mostly human-caused, mostly natural, or some combination.”
These conclusions are consistent with our findings in another two of our papers that were also published in the last few weeks – in separate peer-reviewed scientific journals and using different independent analyses.
That is, in Katata et al. (2023), we confirmed that the IPCC’s estimate of the extent of urbanization bias in the global temperature data was much too low. Meanwhile, in Connolly et al. (2023), we concluded that it is “unclear whether the observed warming is mostly human-caused, mostly natural or some combination of both”.
Dr. Schmidt and the RealClimate team apparently do not want you to read our papers. They seem to be afraid that if you did, their claims on climate change would no longer seem so convincing.
In contrast, we are not afraid of people reading papers that disagree with us. In fact, we encourage people to read multiple scientific perspectives and form their own opinions. We agree with J.S. Mill’s quote below:
Below are links to the papers we mentioned above. We look forward to further discussion on our papers
References mentioned
- W. Soon, R. Connolly, M. Connolly, S.-I. Akasofu, S. Baliunas, J. Berglund, A. Bianchini, W.M. Briggs, C.J. Butler, R.G. Cionco, M. Crok, A.G. Elias, V.M. Fedorov, F. Gervais, H. Harde, G.W. Henry, D.V. Hoyt, O. Humlum, D.R. Legates, A.R. Lupo, S. Maruyama, P. Moore, M. Ogurtsov, C. ÓhAiseadha, M.J. Oliveira, S.-S. Park, S. Qiu, G. Quinn, N. Scafetta, J.-E. Solheim, J. Steele, L. Szarka, H.L. Tanaka, M.K. Taylor, F. Vahrenholt, V.M. Velasco Herrera and W. Zhang (2023). “The Detection and Attribution of Northern Hemisphere Land Surface Warming (1850–2018) in Terms of Human and Natural Factors: Challenges of Inadequate Data”, Climate, 11(9), 179; https://doi.org/10.3390/cli11090179. (Open access)
- R. Connolly, W. Soon, M. Connolly, S. Baliunas, J. Berglund, C.J. Butler, R.G. Cionco, A.G. Elias, V. Fedorov, H. Harde, G.W. Henry, D.V. Hoyt, O. Humlum, D.R. Legates, N. Scafetta, J.-E. Solheim, L. Szarka, V.M. Velasco Herrera, H. Yan and W.J. Zhang (2023). “Challenges in the detection and attribution of Northern Hemisphere surface temperature trends since 1850”. Research in Astronomy and Astrophysics. https://doi.org/10.1088/1674-4527/acf18e. (Still in press, but pre-print available here)
- G. Katata, R. Connolly and P. O’Neill (2023). “Evidence of urban blending in homogenized temperature records in Japan and in the United States: implications for the reliability of global land surface air temperature data”. Journal of Applied Meteorology and Climatology. 62(8), 1095-1114. https://doi.org/10.1175/JAMC-D-22-0122.1. (Open access)
- R. Connolly, W. Soon, M. Connolly, S. Baliunas, J. Berglund, C. J. Butler, R. G. Cionco, A. G. Elias, V. M. Fedorov, H. Harde, G. W. Henry, D. V. Hoyt, O. Humlum, D. R. Legates, S. Lüning, N. Scafetta, J.-E. Solheim, L. Szarka, H. van Loon, V. M. Velasco Herrera, R. C. Willson, H. Yan and W. Zhang (2021). “How much has the Sun influenced Northern Hemisphere temperature trends? An ongoing debate”. Research in Astronomy and Astrophysics, 21, 131. https://doi.org/10.1088/1674-4527/21/6/131. (Open access)
- M.T. Richardson and R.E. Benestad (2022). “Erroneous use of Statistics behind Claims of a Major Solar Role in Recent Warming”. Research in Astronomy and Astrophysics, 22, 125008. http://dx.doi.org/10.1088/1674-4527/ac981c. (pdf available here).
- N. Scafetta, R.C. Willson, J.N. Lee and D.L. Wu (2019). “Modeling Quiet Solar Luminosity Variability from TSI Satellite Measurements and Proxy Models during 1980–2018”. Remote Sensing. 11(21), 2569. https://doi.org/10.3390/rs11212569. (Open access)
- Montillet, J.-P., Finsterle, W., Kermarrec, G., Sikonja, R., Haberreiter, M., Schmutz, W., & Dudok de Wit, T. (2022). “Data fusion of total solar irradiance composite time series using 41 years of satellite measurements”. Journal of Geophysical Research: Atmospheres, 127, e2021JD036146. https://doi.org/10.1029/2021JD036146. (Preprint here)
- S. Dewitte and S. Nevens (2016). “The Total Solar Irradiance Climate Data Record”. The Astrophysical Journal, 830 25. https://doi.org/10.3847/0004-637X/830/1/25 (Open access).
- T. Dudok de Wit, G. Kopp, C. Fröhlich and M. Schöll (2017). “Methodology to create a new total solar irradiance record: Making a composite out of multiple data records”. Geophysical Research Letters, 44(3), 1196-1203. https://doi.org/10.1002/2016GL071866. (Open access)
- K. Matthes, B. Funke, M.E. Andersson, L. Barnard, J. Beer, P. Charbonneau, M.A. Clilverd, T. Dudok de Wit, M. Haberreiter, A. Hendry, C.H. Jackman, M. Kretzschmar, T. Kruschke, M. Kunze, U. Langematz, D.R. Marsh, A.C. Maycock, S. Misios, C.J. Rodger, A.A. Scaife, A. Seppälä, M. Shangguan, M. Sinnhuber, K. Tourpali, I. Usoskin, M. van de Kamp, P.T. Verronen and S. Versick (2017) “Solar forcing for CMIP6 (v3.2)”. Geoscientific Model Development, 10, 2247–2302. https://doi.org/10.5194/gmd-10-2247-2017 . (Open access).
- N. A. Krivova, L. Balmaceda and S. K. Solanki (2007). “Reconstruction of solar total irradiance since 1700 from the surface magnetic flux”. Astronomy and Astrophysics, 467, 335-346. https://doi.org/10.1051/0004-6361:20066725 . (Open access).
- O. Coddington, J.L. Lean, P. Pilewskie, M. Snow and D. Lindholm (2016). “A Solar Irradiance Climate Data Record”. Bulletin of the American Meteorological Society, 97(7), 1265-1282. https://doi.org/10.1175/BAMS-D-14-00265.1 . (Open access)
- S. Dewitte, J. Cornelis and M. Meftah (2022). “Centennial Total Solar Irradiance Variation”, Remote Sensing, 14(5), 1072. https://doi.org/10.3390/rs14051072 . (Open access)
- T. Egorova, W. Schmutz, E. Rozanov, A.I. Shapiro, I. Usoskin, J. Beer, R.V. Tagirov and T. Peter (2018). “Revised historical solar irradiance forcing”. Astronomy and Astrophysics, 615, A85. https://doi.org/10.1051/0004-6361/201731199 (Open access)
- D.V. Hoyt and K.H. Schatten (1993). “A discussion of plausible solar irradiance variations, 1700-1992”. Journal of Geophysical Research Space Physics, 98(A11), 18895-18906. https://doi.org/10.1029/93JA01944 . (pdf available here)
- V. Penza, F. Berrilli, L. Bertello, M. Cantoresi, S. Criscuoli and P. Giobbi (2022). “Total Solar Irradiance during the Last Five Centuries”. The Astrophysical Journal, 937(2), 84. https://doi.org/10.3847/1538-4357/ac8a4b . (Open access).
- W. W.-H. Soon (2005). “Variable solar irradiance as a plausible agent for multidecadal variations in the Arctic-wide surface air temperature record of the past 130 years”. Geophysical Research Letters. 32, 16. https://doi.org/10.1029/2005GL023429 (Open access)
- W. W.-H. Soon (2009). “Solar Arctic-Mediated Climate Variation on Multidecadal to Centennial Timescales: Empirical Evidence, Mechanistic Explanation, and Testable Consequences”. Physical Geography. 30, 144-184. https://doi.org/10.2747/0272-3646.30.2.144 (pdf available here).
- W. Soon, Ronan Connolly and M. Connolly (2015). “Re-evaluating the role of solar variability on Northern Hemisphere temperature trends since the 19th century”. Earth-Science Reviews, 150, 409-452. https://doi.org/10.1016/j.earscirev.2015.08.010. (Preprint version)
- P. O’Neill, R. Connolly, M. Connolly, W. Soon, B. Chimani, M. Crok, R. de Vos, H. Harde, P. Kajaba, P. Nojarov, R. Przybylak, D. Rasol, Oleg Skrynyk, Olesya Skrynyk, P. Štěpánek, A. Wypych and P. Zahradníček (2022). “Evaluation of the homogenization adjustments applied to European temperature records in the Global Historical Climatology Network dataset”. Atmosphere, 13(2), 285. https://doi.org/10.3390/atmos13020285. (Open access)
- W.W-H. Soon, R. Connolly, M. Connolly, P. O’Neill, J. Zheng, Q. Ge, Z. Hao and H. Yan (2018). Comparing the current and early 20th century warm periods in China. Earth-Science Reviews, 185, 80-101. https://doi.org/10.1016/j.earscirev.2018.05.013. (Preprint version)
- G.A. Schmidt, J.H. Jungclaus, C.M. Ammann, E. Bard, P. Braconnot, T.J. Crowley, G. Delaygue, F. Joos, N.A. Krivova, R. Muscheler, B.L. Otto-Bliesner, J. Pongratz, D.T. Shindell, S.K. Solanki, F. Steinhilber, and L.E.A. Vieira (2011). “Climate forcing reconstructions for use in PMIP simulations of the last millennium (v1.0)”. Geoscientific Model Development, 4, 33–45, https://doi.org/10.5194/gmd-4-33-2011. (Open access).