Meta launches speech-to-text, text-to-speech AI models for more than 1,100 languages; even open source data sharing
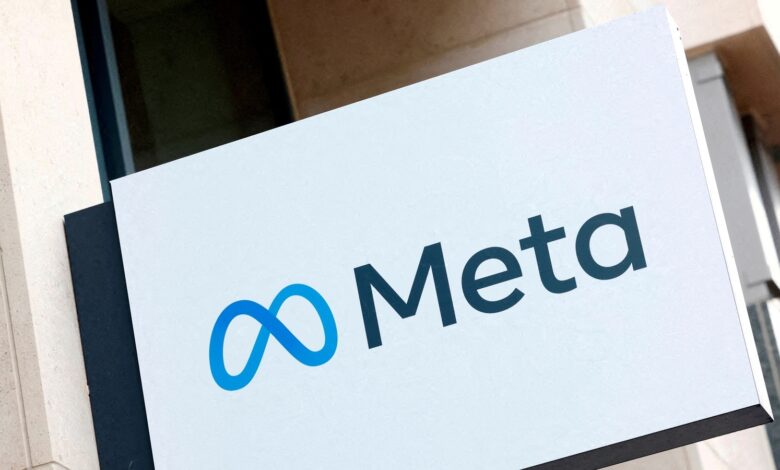
All tech professionals are in a fierce battle to provide convenience to users in the form of artificial intelligence (AI)-enhanced products. While everyone knows about OpenAI’s ChatGPT and Google’s Bard, very little is known about it from Facebook co-founder Mark Zuckerberg’s Meta Platform. As of today, that is. Now, the company has rolled out text-to-speech, text-to-speech AI models for more than 1,100 languages, and the best part is that it’s not affiliated with ChatGPT. Check out the Multilingual Speech (MMS) project.
The most notable point is that Meta has shared open source and that means it can lead to a spike in the number of speeches. application created worldwide.
If all goes well in the real world, how useful this could be given Meta’s statement, “Current speech recognition models only cover about 100 languages — a fraction of the more 7,000 known languages are spoken on the planet.”
Data processing
Now, good machine learning models require large amounts of labeled data — in this case, thousands of hours of audio along with transcripts. For most languages, this data simply does not exist.
However, Meta has overcome that through the MMS project, which combines wav2vec 2.0, its pioneering work in self-supervised learning, and a new dataset that provides labeled data for more than 1,100 languages and unlabelled data for nearly 4,000 languages.
Pampering herself, Meta, in a statement said: “Our results show that Mass Multilingual Speech models outperform existing models and cover 10 times more languages time.”
It also revealed that, “Today, we publicly share our models and code so that others in the research community can build on our work. Through this work, We hope to make a small contribution to preserving the world’s incredible linguistic diversity.”
How did Meta do it?
The first work of the MMS project was to collect audio data for thousands of languages, but the largest speech dataset available includes up to 100 languages. The challenge was overcome by “turning to religious texts, such as the Bible, which have been translated into many different languages and whose translations have been extensively studied for language-based translation studies.” on text”.
The MMS project even produced a dataset of New Testament readings in more than 1,100 languages.
Realizing that the idea was good and that it could be exploited more, the project also looked at unlabelled recordings of many other Christian religious readings. This has increased the number of languages available to more than 4,000.
Bias, what bias?
Even when the data comes from a specific domain, biases don’t seem to be introduced into the system. This is clear from the fact that although this text is generally read by male speakers, Meta analysis shows that its MMS models work equally well for male and female voices.
And importantly, while the content of the audio recordings is religious, the MMS analysis shows that this is not too biased towards the model for generating more religious languages.
Meta attributes this success to the connectionist’s use of the temporal classification method, which is said to be better than large language models (LLMs) or tracing models. sequence for speech recognition.
How it was made usable
Meta preprocessed the data to make it usable by machine learning algorithms by training an alignment model on existing data in more than 100 languages.
To reduce error rates, Meta says: “We applied multiple rounds of this process and performed a final cross-validation filtering step based on model accuracy to eliminate potentially erroneous data. deviated.
Results
Multilingual speech recognition models are meta-trained on more than 1,100 languages. The consequence of this has been explained by Meta this way, “As the number of languages increases, the performance decreases, but only very slightly: Going from 61 to 1,107 languages only increases the character error rate up. about 0.4 percent but increased the range of languages more than 18 times.”
MMS vs OpenAI Whisper
In a similar comparison with Whisper, Meta says that models trained on Massively Multilingual Speech data only achieve half from error rate, but importantly Mass Multilingual Speech covers 11 times more languages.