Stampede2 supercomputer helps uncover new properties of high entropy alloys
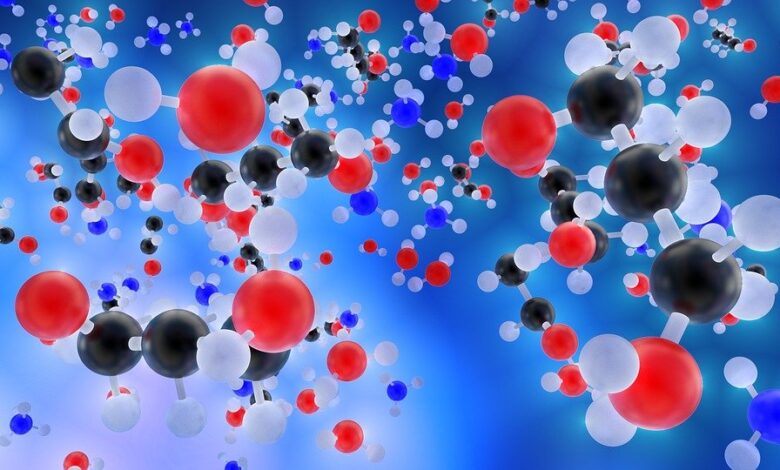
When is something more than just the sum of its parts? Alloy demonstrate such synergy. For example steel revolutionize industrially by taking iron, adding some carbon, and creating an alloy much harder than either of its components.

Image credit: Pixabay (License Pixabay Free)
Supercomputer simulations are helping scientists discover new types of alloys, known as high-entropy alloys. The researchers used Stampede2 Texas Advanced Computing Center (TACC) supercomputer distributed by the Polar Science and Engineering Discovery Environment (XSEDE).
Their research is published in April 2022 at Npj Computational Materials. This approach can be applied to finding new materials for batteries, catalysts and more without the need for expensive metals like platinum or cobalt.
“High entropy alloy represents a completely different design concept. In this case, we try to combine many key factors together,” said the senior author of the study. Wei Chenassociate professor of materials science and engineering at the Illinois Institute of Technology.
The term “high” ChaosIn short, it refers to the energy drop obtained from the random mixing of many elements in the same atomic fractions, which can stabilize new and novel materials from ‘ cocktails.’

Shown is a data-driven workflow for mapping the elastic properties of the high entropy alloy space. Supplier: Chen et al.
For the study, Chen and colleagues surveyed a large space of 14 elements and the combinations that produce high-entropy alloys. They performed high-throughput quantum mechanical calculations, finding the alloys’ stability and elasticity, and their ability to regain their size and shape after stress, of more than 7,000 high-entropy alloys. .
“To our knowledge, this is the largest database on the elastic properties of high entropy alloys,” added Chen.
Then they take this large data set and apply Deep set architecture, is an advanced work study carefully architecture generates predictive models for the properties of new high-entropy alloys.
“We developed a new machine learning model and predicted the properties of more than 370,000 high-entropy alloy components,” said Chen.
The final part of their study uses what is known as association rule mining, a rule-based machine learning method used to discover new and interesting relationships between variables, in In this case, individual ways or combinations of elements will affect the properties of the high entropy alloy.
“We derived several design rules for developing high entropy alloys. And we suggested some compositions that experimentalists could try to synthesize and create,” added Chen.
High entropy alloys are a new frontier for materials scientists. Thus, there are very few experimental results. Thus, this lack of data has limited the ability of scientists to design new ones.
“That’s why we perform high-throughput calculations, to investigate a very large number of high-entropy alloy spaces and understand their stability and elasticity,” said Chen.
He covered more than 160,000 first-principles calculations in this latest work.

Graphs of the bonding rules between the elements and the elastic properties of high-entropy alloys. The results give (a) bulk modulus, (b) Young’s modulus, (c) shear modulus, (d) Pugh ratio, (e) Poisson ratio and (f) Zener ratio respectively. The button colors and sizes represent different elements (as shown in the legend) and fractions. Image supplier: Chen et al.
“The sheer amount of computation is basically impossible to do on clusters of computers or personal computers,” says Chen. “That’s why we need access to high-performance computing facilities, like those at TACC allocated by XSEDE.”
Chen was awarded time on the Stampede2 supercomputer at TACC through XSEDE, a virtual collaboration funded by the National Science Foundation (NSF) to facilitate free, customized access to resources. Advanced digital, consulting, training and mentoring.
Unfortunately EMTO-CPA The Chen code used for quantum mechanical density function theory calculations is inconsistent with the parallel nature of high performance computeroften perform large calculations and split them into smaller calculations that run concurrently.
“Stampede2 and TACC through XSEDE have provided us with a very useful code called Launcher, which helps us pack small jobs into one or two big jobs, so we can get the most out of them. Stampede2’s high-performance computing nodes,” said Chen.
The Launcher The script developed at TACC allows Chen to pack around 60 small jobs into one and then run them concurrently on a high-performance node. That increases their computational efficiency and speed.
“Clearly this is a unique use case for supercomputers, but it is also quite common for many material modeling problems,” says Chen.
For this work, Chen and colleagues applied a computer network architecture called Deep Sets to model the properties of high entropy alloys.
The Deep Sets architecture can use the elemental properties of each individual high-entropy alloy and build predictive models to predict the properties of a new alloy system.
“Because the framework is so efficient, most of the training is done on our students’ personal computers,” says Chen. “However, we used TACC Stampede2 to make predictions using the model.”
Chen gave the example of extensive research Alloy Cantor – an almost equal mixture of iron, manganese, cobalt, chromium and nickel. The cool thing about it is that it doesn’t get brittle at very low temperatures.
One reason for this is what Chen calls the ‘cocktail effect’, which induces surprising behaviors relative to the constituent elements when they are mixed together in roughly equal parts like an entropy alloy. high.
Another reason is that when many elements are mixed, an almost unlimited design space is opened up to search for new component structures and even a completely new material for applications that were not previously available. this cannot be done.
“It is hoped that more researchers will use computational tools to help them narrow down the materials they want to synthesize,” said Chen. “High-entropy alloys can be made from easily derived elements, and hopefully we can replace precious metals or elements like platinum or cobalt that have supply chain problems. response. These are really strategic and sustainable materials for the future.”
Research, “Component design of high entropy alloys with deep learning“Published in April 2022 in Computational Materials Npj. The study authors are Jie Zhang, George Kim and Wei Chen of the Illinois Institute of Technology; Chen Cai and Yusu Wang of the University of California San Diego.
Written by Jorge Salazar
Source: TACC