What an LLM Can Do for Radiologists and the Radiologist Shortage
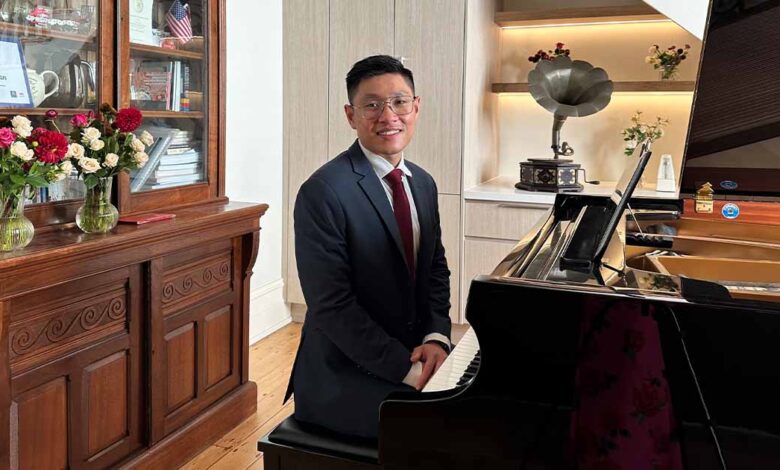
Large language models are rapidly becoming a key building block in new information systems for administrators and clinicians in hospitals and health systems. This form of artificial intelligence can accomplish tasks that no human can imagine.
Harrison.ai develops artificial intelligence technology to speed up clinical diagnosis and offers a suite of AI pathology and radiology tools designed to improve efficiency for physicians to help address burnout.
Healthcare IT News spoke with Dr. Aengus Tran, co-founder and CEO of Harrison.ai, to talk about LLM and radiology: why they fit, what genAI models can do for radiologists, how radiologists can ensure their quality and accuracy — and how applying LLM to radiology can help address the radiologist shortage.
Q. Why are large language models suitable for radiology?
ONE. Large language models have the potential to solve some of radiology’s most pressing challenges. While many AI models that have entered healthcare are only capable of performing predefined tasks, advances in machine learning are increasing the ability of new models to continuously learn and generalize to domains for which they have not been trained.
This is the next transformation of AI in healthcare – an industry where drawing conclusions based on previous experience and knowledge when faced with new and unknown conditions is crucial to providing appropriate care to patients.
The way a radiology LLM is trained is no different than how medical students learn diagnostic radiology – through constant practice, reviewing cases, and studying the literature. A well-trained LLM model should be able to achieve human-level performance on tasks such as analyzing radiographic images for abnormalities, localization, comparison with previous data, and prediction of outcomes.
LLM can provide direct and immediate benefits for radiologists, as it helps them deal with the rapidly expanding medical data landscape by quickly processing and integrating information from multiple sources.
Whether interpreting text data such as medical documents and patient histories or analyzing visual image data, these models can provide radiologists with comprehensive insights that previously required significant time and resources to compile.
Additionally, since X-ray images are digitized, there is a wealth of high-quality standardized data that is unique in the field and suitable for AI intervention.
Q. What can an LLM degree do for a radiologist?
ONE. Healthcare facilities around the world are struggling with the increasing volume of medical images and associated data per patient, a shortage of radiologists, and the risk of physician burnout.
A radiology LLM program can rapidly process medical information, patient histories, and imaging data, potentially providing radiologists with comprehensive insights in a fraction of the time.
Additionally, LLM can provide diagnostic decision support for radiologists by interpreting imaging data, identifying abnormalities, suggesting possible diagnoses, and automating time-consuming administrative tasks. Radiologists can then make faster and more accurate decisions, allowing them to see more patients while reducing their overall workload.
Contrary to initial concerns about AI replacing radiology jobs, LLMs – or at least the way we see them evolving – are not intended to replace human expertise but to enhance and extend it.
Although many LLM programs around the world are strong, they are broad and general in focus.
These general models are not suitable for a domain that is completely dependent on accuracy and has no tolerance for errors. A specialized and nuanced function like healthcare requires a specialized model.
Q. How can a radiologist be assured of the quality and accuracy of the work an LLM is doing for them? How can they feel comfortable?
ONE. A model is only as good as the data it is trained on – and we need to be sensitive to the risks and challenges associated with using LLMs. The effectiveness of LLMs depends on three key factors in their training data: quality, volume, and variety. By leveraging datasets that excel in these aspects, we can create sophisticated systems capable of producing accurate and high-quality output.
Furthermore, comprehensive evaluation is essential. Evaluating LLMs for use in radiology comes with additional challenges – to evaluate basic models, we must move to a model where we test them for their ability to recognize individual pathologies and their overall radiological interpretation skills.
This means that more rigorous testing of the safety and accuracy of LLMs is required. This includes testing against international standards and benchmarks, comparing performance between LLM in industry and put models to practical evaluation.
Several benchmarks have been proposed to evaluate and compare the performance of multimodality platform models on medical tasks. Our view is that LLMs should be tested not only against these benchmarks but also against examinations performed by radiologists, who are considered the gold standard when it comes to medical image interpretation.
This rigorous evaluation process serves two purposes: Building trust among radiologists by demonstrating the model’s comprehensive authenticity while also establishing the model’s legitimacy as a reliable assistive technology.
Q. How can an LLM in radiology help address the radiologist shortage?
ONE. Global healthcare is facing many intersecting challenges, including increased imaging volumes and associated data for each case, shortages of healthcare professionals, and the risk of burnout for remaining staff. The LLM has the potential to help address these issues by improving productivity and efficiency in diagnostic processes:
-
They can improve the efficiency of manual data annotation to generate large labeled datasets for comprehensive medical imaging AI.
-
They can enable easy access and search of cases by analyzing radiology reports, thus facilitating rapid, efficient and continuous quality assessment.
-
Importantly, as a model that can operate anywhere, at any time of day, LLM can facilitate better access to radiology services in remote and underserved areas. This means providing primary and supportive readings to clinicians who may be working in isolated locations or under-resourced settings, improving equitable access to timely and accurate diagnoses for patients around the world.
Most of these activities are time-consuming but can be streamlined with AI, allowing radiologists to focus on the critical decision-making elements of their work that have the highest impact on patient care.
Follow Bill’s HIT coverage on LinkedIn: Bill Siwicki
Email him: [email protected]
Healthcare IT News is a publication of HIMSS Media.
The HIMSS Healthcare AI Forum is scheduled for September 5-6 in Boston. Learn more and register.